Impact of 30 years precipitation regime differences on forest soil physiology and microbial assemblages
- 1EVA4.0 Unit, Faculty of Forestry and Wood Sciences, Czech University of Life Sciences Prague, Prague, Czechia
- 2Faculty of Forestry and Wood Sciences, Department of Genetics and Physiology of Forest Trees, Czech University of Life Sciences Prague, Prague, Czechia
- 3Excelentní Tým Pro Mitigaci (ETM), Faculty of Forestry and Wood Sciences, Czech University of Life Sciences Prague, Prague, Czechia
- 4Faculty of Science, BIOCEV, Charles University, Prague, Czechia
- 5Institute of Microbiology of the Czech Academy of Sciences, Prague, Czechia
Anthropogenic disturbances and climate change affect abiotic and biotic environmental drivers in forest ecosystems. Global warming impacts the soil moisture content, thus influencing the diversity, abundance and functioning of soil microfauna. However, limited studies have been conducted to evaluate the impact of long-term variation in precipitation on soil microfauna. A better understanding of soil microfauna functioning under various precipitation regimes can aid in formulating better prediction models for assessing future climate change impacts. The present study uses a multi-omics approach to capture the variability in the total organic carbon (TOC), total nitrogen (TN), pH, metabolites, microbial biomass and function due to the difference in soil water content mediated by long-term precipitation (30 years) and soil texture differences in two Norway spruce seed orchards. Results showed a positive correlation between TOC, TN, extracellular enzyme activities (EEAs) and phospholipid fatty acids (PLFA) content with higher precipitation, whereas microbial diversity showed an opposite trend. A distinct metabolic profile was observed between the two forest soils. Furthermore, variance partitioning canonical correspondence analysis (VPA) revealed a higher contribution of TOC and TN in shaping the microbial communities than soil pH and conductivity in Norway spruce seed orchards. Our study generates field data for modeling the impact of long-term precipitation variance supplemented by soil texture on soil microbial assemblage and function in Norway spruce stands.
Highlights
- Limited studies have been conducted to evaluate the impact of long-term variation in precipitation on forest soil microfauna.
- Multi-omics approach to capture the variability in forest soil quality parameters and microbial community composition due to long-term precipitation differences over three decades.
- A positive correlation existed between TOC, TN, extracellular enzyme activities (EEAs), and phospholipid fatty acids (PLFA) content with higher precipitation.
- Microbial diversity showed a negative correlation with precipitation.
- Long-term precipitation difference alters soil metabolomic profile towards more acid and alcohol derivatives.
1. Introduction
Soil is an integral component of the forest ecosystem, which presently comprises an estimated 38–40 million square kilometers on Earth or 30% of the total global land area (Baldrian, 2017; Lladó et al., 2017). Forest soils, in particular, are spatially and temporally heterogeneous. They are characterized by strong physicochemical gradients (Huhta and Setälä, 1990), offering mosaic microhabitats for a diverse spectrum of microorganisms, such as bacteria, archaea, and fungi. Diverse soil microbial communities influence forest health and productivity and thus help maintain its long-term sustainability (Micallef et al., 2009; Chernov et al., 2021; Mishra et al., 2022). Precisely, microbes contribute to soil organic matter (SOM) processing, organic C turnover and sequestration, orchestration of the biogeochemical cycling of various essential elements (i.e., nitrogen, phosphorus, sulfur), and contribute to soil structure formation and stabilization (Banerjee and Chapman, 1996; Li et al., 2015; Fang et al., 2016; De Feudis et al., 2017; He et al., 2017; Martinoviæ et al., 2022). Besides, microbes also improve plant growth, fitness, and resistance by facilitating nutrient uptake and thus contribute to forest ecosystem productivity and fertility (Uroz et al., 2013; Li et al., 2015; Chodak et al., 2016; Chang et al., 2017; Haas et al., 2018). Soil microbes bridge the gap between above and belowground processes in forests and significantly shape the structure of the whole ecosystem (Chang et al., 2017).
The size, composition, and activity of soil microbial communities rely on biotic and abiotic factors. Several studies have revealed a strong correlation between the structural and functional diversity of soil microbial communities based on specific tree species composition (Li et al., 2014; Chodak et al., 2016), amount of litter, and soil substrate quality (el Zahar Haichar et al., 2008; Krashevska et al., 2015; He et al., 2017). Soil texture, moisture, and temperature are among the most crucial environmental drivers impacting microbial biomass (De Feudis et al., 2017). Soil texture plays a vital role in carbon storage and strongly affects nutrient retention and availability in forest ecosystems (Silver et al., 2000). Clay-rich soil tends to accumulate organic carbon more rapidly than sandy soil and delays the decomposition of the organic matter (Six et al., 2000, 2002). In addition, soil texture also influences soil moisture, which impacts organic carbon accumulation by manipulating the quantity of carbon input from plants and their decomposition rate in soil (Zhou et al., 2008; O’brien et al., 2010). Similarly, soil pH may have the most decisive influence in the case of bacteria due to the higher sensitivity of bacterial cells to narrow pH alterations (Fierer and Jackson, 2006; Rousk et al., 2010; Chaparro et al., 2012; Lladó et al., 2017). Soil pH presumably also influences the bacterial/fungal ratio as soil acidification can reduce the bacterial abundance and favor fungal predominance (Coûteaux et al., 1998; Kennedy and Maillard, 2022). This phenomenon can be observed in acidic coniferous soils, where fungal biomass tends to exceed the bacterial. Alternatively, elevated deposition of atmospheric N causes a shift from fungal predominance to bacterial (Berg et al., 1998; Coûteaux et al., 1998; Gao et al., 2016; Frey et al., 2020). Alterations in soil microbial community composition may affect the degradation of soil organic matter and other physiological processes in soil by secreting a diverse set or number of enzymes based on varied microbial abundance (Sinsabaugh, 2010; Fang et al., 2016; Borowik et al., 2022; Wu et al., 2022). Hence, amendments in the soil microbial equilibrium affect the energy and nutrients flow within the forest ecosystem and impact the aboveground life.
Although the importance of soil microbiome within forest ecosystems is widely recognized (Lladó et al., 2017; Naylor et al., 2020; Li et al., 2022), limited studies are conducted using state-of-the-art omics approaches. Soil microbial diversity and assemblages are understated in most of the studies in the field so far (Huang et al., 2015; Zhou et al., 2017; Maxwell et al., 2020). Even the existing omics-based studies mainly dealt with bacteria or fungi (Yuste et al., 2011; Zhang et al., 2013; Wang et al., 2014; Li et al., 2016; Zhang et al., 2017; Anthony et al., 2022). Furthermore, the multi-omics approach to gaining in-depth insight is still rare in soil microbial studies (Hultman et al., 2015; Bastida et al., 2017; Overy et al., 2021; Yang et al., 2021). Hence, the current knowledge about the true response of the soil microbial community to edaphic and climatic factors is not adequate and somewhat inconsistent, i.e., the impact of drought on soil microbial biomass is contradictory between independent study outcomes (Manzoni et al., 2012; Barnard et al., 2013; De Vries and Shade, 2013; Rousk et al., 2013; Canarini et al., 2016).
Nonetheless, alterations in climate conditions and disturbances will affect both abiotic and biotic environmental drivers (Bowd et al., 2022; Meena et al., 2022). Perhaps water availability is crucial in governing soil microbial respiration and their feedback response to climate change (Liu et al., 2016; Bowd et al., 2022). Global warming induces a shift in the global precipitation regime that substantially influences the microbial community function and their services to the ecosystem (Knapp et al., 2017; Wilcox et al., 2017). To be precise, changes in precipitation and warming of the soil are expected to alter the microbial community composition, the ratio of bacteria and fungi, and their functions. For instance, studies showed that microbial activity is directly influenced by osmotic stress or limited substrate diffusion during short-term drought (Schimel, 2018).
In contrast, long-term droughts modify soil microbial community composition and subsequently alter the nutrient flow and strategies for C utilization (Fang et al., 2016; Su et al., 2020; Sridhar et al., 2022). Alternatively, higher precipitation can influence microbial activity via increasing diffusion of soluble C substrates (Hungate et al., 2007; Yan et al., 2011), enhancing plant growth and C allocation (Zak et al., 1993; Zak et al., 1994). It is worth mentioning that the positive correlation between water availability and microbial activity is valid until a threshold level, but above that, higher water availability affects microbial activity by reducing oxygen concentration (Linn and Doran, 1984; Horz et al., 2004). Hence, accurate assessment and future prediction of the C budget demand a better understanding of the impact of diverse precipitation levels on microbe-mediated belowground processes (Nielsen and Ball, 2015; Schimel, 2016).
Over the last decades, experiments based on altered precipitation have risen substantially (Zhou et al., 2017). Although meta-analysis studies successfully linked the impact of altered precipitation with belowground microbial community structure and function but failed to provide a comprehensive understanding of the magnitude and direction of the effect varied based on other climatic factors (Zhou et al., 2017). Unfortunately, most of the studies were conducted for a brief period of precipitation alteration, which failed to capture the effect of the long-term difference in seasonal precipitation on the forest soil microbial community structure and function. Hence, studies on the impact of long-term precipitation on soil physiology, microbial community structure, and function are of the utmost importance in fulfilling the existing knowledge gap and formulating better prediction models for assessing climate change impacts (Zhang et al., 2017). Furthermore, due to the recent boom in omics technology, it is now possible to apply a multi-omics approach within a single experimental study (Hultman et al., 2015; Bastida et al., 2016; Yang et al., 2021) and get an overview of the belowground bacterial and fungal species composition and function, which is rare in most of the previously conducted studies.
Hence, the present study aims to expand the current knowledge on the impact of the long-term influence of high precipitation on Norway spruce forest soil physiology and microbiome using the multi-omics approach. This study is conducted in the experimental forest locations that are just 25 km apart (air-distance) with remarkably different precipitation levels for a long time (over 30 years) with a temperature difference of approximately 1.6°C, probably due to altitude difference (∼400 m). We compared soil physiology (including soil texture), microbial biomass and enzyme activity, metabolic profile, and microbial diversity between forest sites to capture the long-term precipitation-mediated alterations in soil physiology, microbial structure, and function in an unbiased manner. Our findings exposed the long-term impact of precipitation differences complemented by soil texture and pH on belowground microbial communities and processes within Norway spruce forest and delivered field data for modeling the soil microbial retort towards future global climate change scenarios.
2. Materials and methods
2.1. Sampling site and soil sampling
The soil samples were collected from two Norway spruce clonal seed orchards located about 25 kms apart in the south-west of Czech Republic, namely Prenet (P) (49.2354172N, 13.2112808E, 970 m above sea level) and Lipova Lhota (L) (49.2816108N, 13.5515606E, 560 m above sea level) in Oct–Nov 2019. The Prenet site had a north-southern facing slope of 14% with 6 ×6 m spacing of trees, while the Lipova site had tree spacing of 5 × 5 m and a 9% slope facing north-south. Over three decades, the average annual temperature and precipitation recorded were 7.04°C and 1306.48 mm for P- site and 8.64°C and 633 mm for L- site. The soil samples were collected at a depth of 10 cm from the soil surface using a root corer (Eijkelkamp, Netherlands). A total of six randomly selected soil samples from Prenet seed orchard (P-site) and nine replicates from Lipova seed orchard (L-site) were sampled to negate the differences in the land area (L-site>P-site) between the two seed orchards. Altogether 15 soil samples were collected in sterile plastic ziplock bags and brought to the laboratory for the downstream studies. The soil samples were sieved through a 2.0 mm screen sieve, and some of the soil samples were stored at 4°C until the soil physicochemical and biochemical properties were measured. The remaining soil samples were stored at −80°C for PLFA analysis, soil metabolite profiling, and DNA extraction to determine microbial community composition using the next-generation sequencing method. The determination of the physicochemical properties of the samples was performed with air-dried soils, while soil enzyme activities were performed with the field moist soil samples. The results were expressed based on the dry weight of the soil.
2.2. Soil texture and soil water content determination
The soil particle size distribution was determined by laser granulometer (CILAS 1190 LD) to measure the particle size ranging from 0.04 to 2,500 μm in wet mode. The soil samples were pre-treated, as described earlier (Lisá et al., 2017). The soil texture is reported based on three fractions, clay (>2 μm), silt (2–63 μm), and sand (63–2,000 μm) (Wentworth, 1922). The soil water content (SWC, %) was determined by oven drying 5 g of soil at 105°C for 24 h. The results were expressed based on the dry weight of the soil.
2.3. Soil physicochemical properties
The soil physicochemical properties, such as soil pH, electrical conductivity (EC), total organic carbon (TOC), and total nitrogen (TN), were measured using standard procedures. The soil pH (1:5 H2O, w/v) was measured using an ISFET electrode (Sentron, Netherlands). The total organic carbon (TOC) was determined by the dry oxidative combustion method at 1,250°C using a TOC analyzer (SSM-5000A; Shimadzu Corp., Kyoto, Japan) (Nelson and Sommers, 1983). The Kjeldahl method determined the total nitrogen content (TN) in the soil samples (Bremner, 1996). Statistical analysis for significance was performed by the Kruskal–Wallis test.
2.4. Soil enzyme activities
A total of seven hydrolytic soil extracellular enzymes were measured using microplate-based fluorometric and photometric assays described by Baldrian (2009). The enzymes cellobiohydrolase, β-galactosidase, α- glucosidase, β- glucosidase, β-xylosidase are involved in the degradation of organic carbon, while chitinase and acid phosphatase catalyze nitrogen and phosphorous transformation in the soil, respectively (Bell et al., 2013). The moist field soil (1 g) was suspended in 100 ml of distilled water and sonicated for 4 min. The soil suspensions (200 μl) were added to methylumbelliferyl (MUF, pH 7.0) substrate solution (50 μl) in a 96-well plate and incubated at 40°C. After 5 min and 125 min, the fluorescence was recorded using a fluorescence reader (Infinite, TECAN, Austria) with the excitation wavelength 355 nm and emission wavelength 460 nm (Baldrian, 2009). The standard curve was prepared using serial dilutions of the 4-methylumbelliferone (MUF) (Vepsäläinen et al., 2001). Each enzyme activity was determined from the fluorescent values and the standard curve and expressed as nmol g–1 soil h–1 after dry weight correction.
2.5. Phospholipid fatty acids (PLFA)
Phospholipid fatty acids analysis determined the spatial soil microbial community structure (Zelles, 1999) at the two different sites. The PLFAs were extracted from 1 g of freeze-dried soil using a mixture of chloroform-methanol-phosphate buffer (1:2:0.8), as previously described (Stella et al., 2015). The extracted PLFAs were purified using an extraction cartridge (LiChrolut Si-60, Merck, White House Station, NJ, USA) and subjected to mild alkaline methanolysis as mentioned in the earlier report (Šnajdr et al., 2008). The free methyl esters of PLFAs were then analyzed by gas chromatography-mass spectrometry (450-GC, 240-MS ion trap detector, Varian, Walnut Creek, CA, USA) and processed as described earlier (Stella et al., 2015). The fungal biomass in the soil samples was determined based on 18:2ω6,9 fatty acid content, while the bacterial biomass was estimated as the sum i14:0, i15:0, a15:0, 16:1ω5, 16:1ω7; 16:1ω9, 10Me-16:0, i16:0, i17:0, a17:0, cy17:0, 17:0, 10Me-17:0, 18:1ω7, 10Me-18:0, 15:0, and cy19:0. Actinobacteria biomass was quantified according to 10Me-16:0, 10Me-17:0, and 10-Me18:0. The total microbial biomass was determined from the sum of all PLFAs molecules. Different microbial ratios such as fungal: bacteria biomass, actinobacteria: bacteria, and gram-positive bacteria: gram-negative bacteria were also calculated (Moore-Kucera and Dick, 2008).
2.6. Soil metabolite profiling
The extraction of soil metabolites was performed as described previously (Song et al., 2020). The freeze-dried soil samples were homogenized using mortar and pestle and stored at −80°C until extraction. Homogenized soil (500 mg) was added to 600 μl of methanol: H2O, 3:1 (v/v) mixture, and 600 μl ethyl acetate along with 10 μl of adonitol [0.5 mg/ml, internal standard A (IS_A)]. The samples were then sonicated using an ultrasonic rod homogenizer (30 s, 50%, 30 kHz) (Sonoplus mini20, Bandelin). The mixture was incubated in a thermoshaker for 15 min at 10°C and 2,000 rpm, followed by centrifugation at 16,000 g for 15 min at 4°C. The supernatant was transferred into a new tube, and the steps were repeated with the soil pellet. All the supernatants were combined and vacuum dried without heating using a vacuum concentrator (Modul 4080C, Hanil Science Industrial). The samples were then resuspended in 50 μl of anhydrous pyridine and 50 μl of methoxyamine hydrochloride in pyridine (25 mg/ml) and incubated in a thermoshaker at 40°C for 90 min at 1,700 rpm). To this solution, 100 μl of N,O-Bis(trimethylsilyl)trifluoroacetamide with trimethylchlorosilane (BSTFA+TMSC) was added, and the content was incubated in the Thermomixer at 40°C for 30 min. Finally, 10 μl of 1-bromoeicosane [internal standard B (IS_B)] (0.52 mg/ml in hexane) was added, vortexed to mix, and centrifuged (3,000 rpm, 5 min). Finally, the supernatant was carefully transferred and injected into the GCxGC-MS.
The samples were analyzed using a two-dimensional comprehensive gas chromatography with mass detection (GCxGC-MS; Pegasus 4D, Leco Corporation) controlled by ChromaTOF v4.5. The gas chromatograph is coupled with a time-of-flight mass spectrometer (GC-TOF-MS, Pegasus 4D, Leco corporation). A combination of non-polar and polar separation columns was used for the GCxGC analyses: Primary column; Rxi-5SilMS (29.5 m × 0.25 mm, Restek); Secondary column BPX-50 (1.44 m × 0.1 mm, SGE). Other parameters were set as follows: inlet temperature 300°C, injection volume 1 μl in split 10 mode, constant He flow 1 ml/min, modulation time 3 s (hot pulse 1 s), modulation temperature offset to the secondary oven 15°C, transfer line temperature 280°C, ion source temperature 220°C, mass range m/z 85–1,000. Temperature program applied to the primary oven: 50°C (hold 1 min) with a gradual increase to 190°C (8°C/min) followed by an increase to 220°C (4°C/min) and then to 320°C (8°C/min) with 12 min hold. The same program was applied to the secondary oven with a temperature offset of +5°C. Two-dimensional chromatograms of the analyses were aligned and processed in Statistical Compare, an in-build module of ChromaTOF v4.5 software. Metabolites were analyzed as trimethylsilyl derivatives and normalized according to the weight taken to the extraction and internal standards IS_A and IS_B. The metabolites were identified by comparing their mass spectra with those available in NIST Library, Fiehn Library, and the in-house-built mass library. If available in the mass databases, retention indices were determined using linear hydrocarbons with the retention indexes (based on linear hydrocarbons). A sparse PLS discriminant plot was generated based on the sPLS-DA algorithm (Lê Cao et al., 2011). The statistical evaluation of the normalized data was performed using a web-designed platform, MetaboAnalyst 5.01, following Chong et al. (2019). The Euclidean distance measure was applied to cluster the soil samples, and a Ward Clustering Algorithm was used to group the metabolites. The test for significance for all the metabolites present in the soils was performed using a T-test and analysis of variance (ANOVA).
2.7. DNA extraction, amplification, and sequencing
The soil DNA was extracted from the different biological replicates from the two sites and stored at −80°C. About 250 mg of soil samples were used for DNA extraction using the Nucleospin soil DNA purification kit (Macherey Nagel, Germany), following the manufacturer’s protocol. The extracted soil DNA was quantified on Qubit 2.0 Fluorometer using a Qubit 2.0 High sensitivity dsDNA assay kit and electrophoresed on 1% agarose gel to check the DNA integrity. Finally, the isolated soil DNA samples were sent to the Novogene company (China) for high-throughput amplicon sequencing.
The amplicon sequencing was performed using the standardized protocol at the Novogene company. The purified DNA (1 ng/μl) was used for amplification with a specific set of universal primers with unique barcodes targeting the bacterial 16S region (341F/806R) (Klindworth et al., 2013) and the fungal ITS2 domain (ITS3, ITS4) (White et al., 1990). The PCR reactions were performed using Phusion High-Fidelity PCR Master Mix (New England Biolabs). The negative control (no template DNA control) was also included in the reaction. Before the library preparation, the equi-density of PCR amplicons was pooled and gel purified (Qiagen Gel Extraction Kit, Germany). The sequencing library with index codes was prepared using NEBNext Ultra DNA Library Pre-Kit from Illumina. After library preparation, it was quantified in Qubit 2.0 Fluorometer (Thermo Scientific), and the quality of the prepared sequencing library was determined in Agilent Bioanalyser 2100 system. Additionally, a no-template sequencing control was included in the experiment in which the reagents were sequenced without adding the template DNA. The sequencing was performed using Illumina Novaseq 6000 platform to generate 250 bp paired-end reads.
2.8. Sequencing data analysis
2.8.1. Data filtering
The paired-end reads generated from Illumina sequencing were assembled using FLASH (V1.2.7)2 (Magoè and Salzberg, 2011) after the removal of the barcode and primer sequence. High-quality clean tags were obtained after quality filtering of the assembled reads based on pre-set parameters (Bokulich et al., 2013) in QIIME (V1.7.0)3 (Caporaso et al., 2010). Finally, the chimaera sequences were detected using UCHIME algorithm4 (Edgar et al., 2011) by comparing with the respective reference databases, i.e., SILVA database for bacterial 16S sequences5 (Wang et al., 2007), and UNITE database for fungal ITS sequences (Nilsson et al., 2019). The effective Tags were obtained after removing the chimeric sequences (Haas et al., 2011).
2.8.2. Operational taxonomic unit (OTU) analysis
Operational taxonomic unit clustering was performed using UPARSE software (UPARSE v7.0.1001)6 (Edgar, 2013). All sequences with a threshold ≥97% similarity were assigned to the same OTU. Each OTU was then searched for the representative species annotation against the respective reference databases. Bacterial species annotation was done against the SILVA database (Release v138.1) (see text footnote 5) (Wang et al., 2007), while the UNITE database was used for the annotation of fungal species (Nilsson et al., 2019). The phylogenetic relationship of different OTUs was explored by the Multiple sequence alignment using MUSCLE software (Version 3.8.31)7 (Edgar, 2004). Additionally, the singletons obtained during the analysis were removed, and the normalized OTU abundance table was calculated using the number of sequences corresponding to the sample with the lowest reads.
2.8.3. Alpha diversity
The alpha diversity of bacterial and fungal species was calculated based on the normalized OTU abundance. The alpha diversity indices such as microbial diversity (Shannon, Simpson) and community richness (Chao1, ACE) (Magurran, 1988) along with observed-species and sequence depth (Good’s coverage) (Chao et al., 1988) for the soil samples from two different sites were estimated in QIIME (Version 1.7.0) (Caporaso et al., 2010) and represented using R software (Version 2.15.3; R Core Team, 2013, Vienna, Austria) (R Core Team, 2013).
2.8.4. Beta diversity
The beta diversity representing the variation in microbial diversity (Lozupone et al., 2007) between the soil samples collected from the two different sites was measured in QIIME software (Version 1.7.0) (Caporaso et al., 2010). The dissimilarity coefficient between pairwise samples was estimated using weighted and unweighted UniFrac distance matrices. Non-metric multidimensional scaling (NMDS) analysis was carried out to visualize the complex multidimensional data (Oksanen et al., 2010). The hierarchical clustering, such as Unweighted Pair-group Method with Arithmetic Means (UPGMA) (Lozupone et al., 2011), was performed in QIIME software (Version 1.7.0) (Caporaso et al., 2010) to understand the distance matrix using average linkage. Statistical methodologies such as Multi-response permutation procedure (MRPP) analysis (Cai, 2006), Analysis of Similarity (ANOSIM) (Clarke, 1993), and ADONIS (Anderson, 2001) were used to determine the significant variation of the microbial communities in the soils of two different sites. Furthermore, differences in the microbial species abundance in the soil samples between the two sites were analysed by T-test (D’Argenio et al., 2014) and MetaStats (Paulson et al., 2011). The significance level represented by the p-value and q-value was determined by the permutation method and Benjamini and Hochberg False Discovery Rate (FDR) method, respectively (White et al., 2009). The presence of microbial communities with a significant intra-group variation among the soil samples was determined by linear discriminant analysis Effect Size (LEfSe) using LEfSe software (Segata et al., 2011). The linear discriminant analysis scores (LDA score [log10]) >4 was set as a threshold. The functional prediction using PICRUSt analysis (Phylogenetic Investigation of Communities by Reconstruction of Unobserved States, version 1.0.0) (Douglas et al., 2018) was performed on the bacterial 16S OTU table generated by QIIME (Version 1.7.0) (Caporaso et al., 2010) using Kyoto Encyclopedia of Genes and Genomes (KEGG) (Kanehisa et al., 2012) to predict the abundance of different gene families.
2.9. Environmental association analysis
The relationship between the environmental factors (pH, TOC, TN, conductivity) and the species abundance (alpha diversity) was estimated using the Spearman rank correlation (Algina and Keselman, 1999) and Mantel test (Yang et al., 2007). Additionally, the Canonical correspondence analysis (CCA) was performed in R using the Vegan package (Sheik et al., 2012), an ordination approach based on correspondence analysis combined with multivariate regression analysis. The Canonical correspondence analysis is based on unimodal species-environment relationships to determine the major driving environmental forces that influence the development of certain microbial communities. Furthermore, Variance partitioning canonical correspondence analysis (VPA) is performed to determine the relative contribution of selected environmental variables in explaining the microbial community structure (Peres-Neto et al., 2006). The variation partitioning analysis allows the partition of the beta diversity variation among the environmental and spatial variables and determines whether the drivers controlling the spatial organisation are the same for different organisms (Legendre, 2008). The VPA was performed in R using the “varpart” function in the vegan package. The different components of variance were estimated as described previously (Borcard et al., 1992).
3. Results
3.1. Soil texture, physicochemical properties, and extracellular enzyme activities
The granulometric study to determine the particle size distribution revealed that the Prenet (P) site soil was sandy loam (0.8% clay, 28.3% silt, 70.8% sand), while the Lipova (L) site soil had loamy sand texture (0.6% clay, 24.9% silt, 74.3% sand). The P-site soil had high water content (32%) compared to the L-site soil (9%), which could be explained by the soil texture and increased precipitation (long-term) at the P-site (Table 1). The soil pH (1:5 H2O, w/v) ranged from extremely acidic (P-site soil pH 4.45 ± 0.06) to strongly acidic (L-site soil pH 5.06 ± 0.24) between the two seed orchards (Burt, 2014). However, both the soil samples were non-saline with low soil conductivity (P- site soil EC 0.04 ± 0.001 mS/cm; L-site soil EC 0.03 ± 0.005 mS/cm) (Table 1). Furthermore, substantial differences in the total organic carbon (TOC) and nitrogen (TN) content were observed in the soil samples from these two sites. The P-site with relatively high average annual precipitation (1,306 mm) recorded over the last three decades (Supplementary Figure 1) showed higher amounts of total organic carbon (TOC- 6.79% ± 0.14) and total nitrogen (TN- 0.51% ± 0.01) compared to the L-site soils (TOC-3.27% ± 0.13; TN- 0.25% ± 0.01) (p < 0.05) that experienced significantly low annual precipitation (633 mm) (Table 1 and Supplementary Figure 1). The extracellular enzyme activities in soil documented significant variation between the two sites (Table 1). P-site soil showed significantly higher activity of extracellular enzymes such as cellobiohydrolase (234 ± 0.72 nmol g–1h–1), β-galactosidase (337 ± 0.52 nmol g–1h–1), β-glucosidase (1833 ± 5.00 nmol g–1h–1), α- glucosidase (148 ± 0.57 nmol g–1h–1), β-xylosidase (1853 ± 3.37 nmol g–1h–1), chitinase (499 ± 1.38 nmol g–1h–1), and acid phosphatase (2988 ± 5.10 nmol g–1h–1) compared to L-site (p < 0.05, Kruskal-Wallis test) (Table 1). The higher precipitation in the P-site corresponds to higher amounts of available nutrients (TOC, TN) and influences the soil enzyme activities.
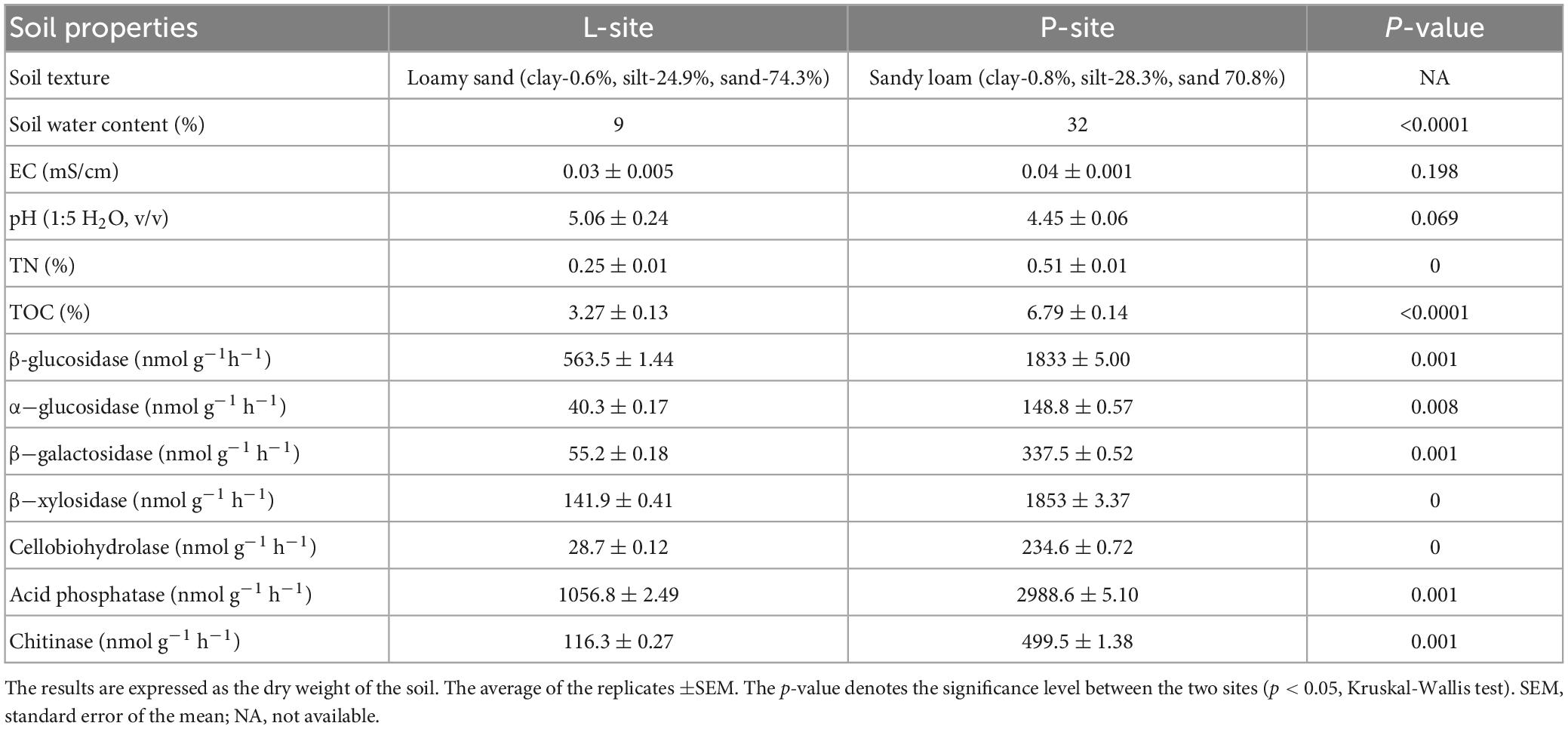
Table 1. Selected physical, physicochemical properties and extracellular enzyme activities in soils from two seed orchards, Lipova (L-site) and Prenet (P-site).
3.2. Soil metabolomics
The high-resolution analysis of the dissolved organic carbon was achieved by soil metabolomics (Swenson and Northen, 2019). The two different soil samples contained 183 metabolites consisting of alcohols, carbohydrates, amino acids, fatty acids and organic acids. Among the total metabolites observed in the soil samples, fatty acids (26.2%) were highly represented, followed by carbohydrates (25.6%), alcohols (12.5%), and organic acids (6.5%) (Supplementary Excel 1). The component analysis revealed that the soil metabolite profile from the two sites was distinct and hence clustered separately (Figure 1A). The top 70 metabolites that were differentially present in the soil samples from two different seed orchards (P and L) are represented in the heatmap (Figure 1B). Prenet soil (P) showed a high abundance of fatty acids, carbohydrates, and alcohols compared to Lipova soil (L). The decomposition of soil organic matter, plant exudates, and microbial metabolites primarily contributed to the overall soil metabolites. The presence of high organic carbon in the Prenet soil corresponds to high amounts of soil metabolites. However, it is worth mentioning here that the contribution of microbial metabolites in the soil is difficult to differentiate between other driving factors (White et al., 2017).
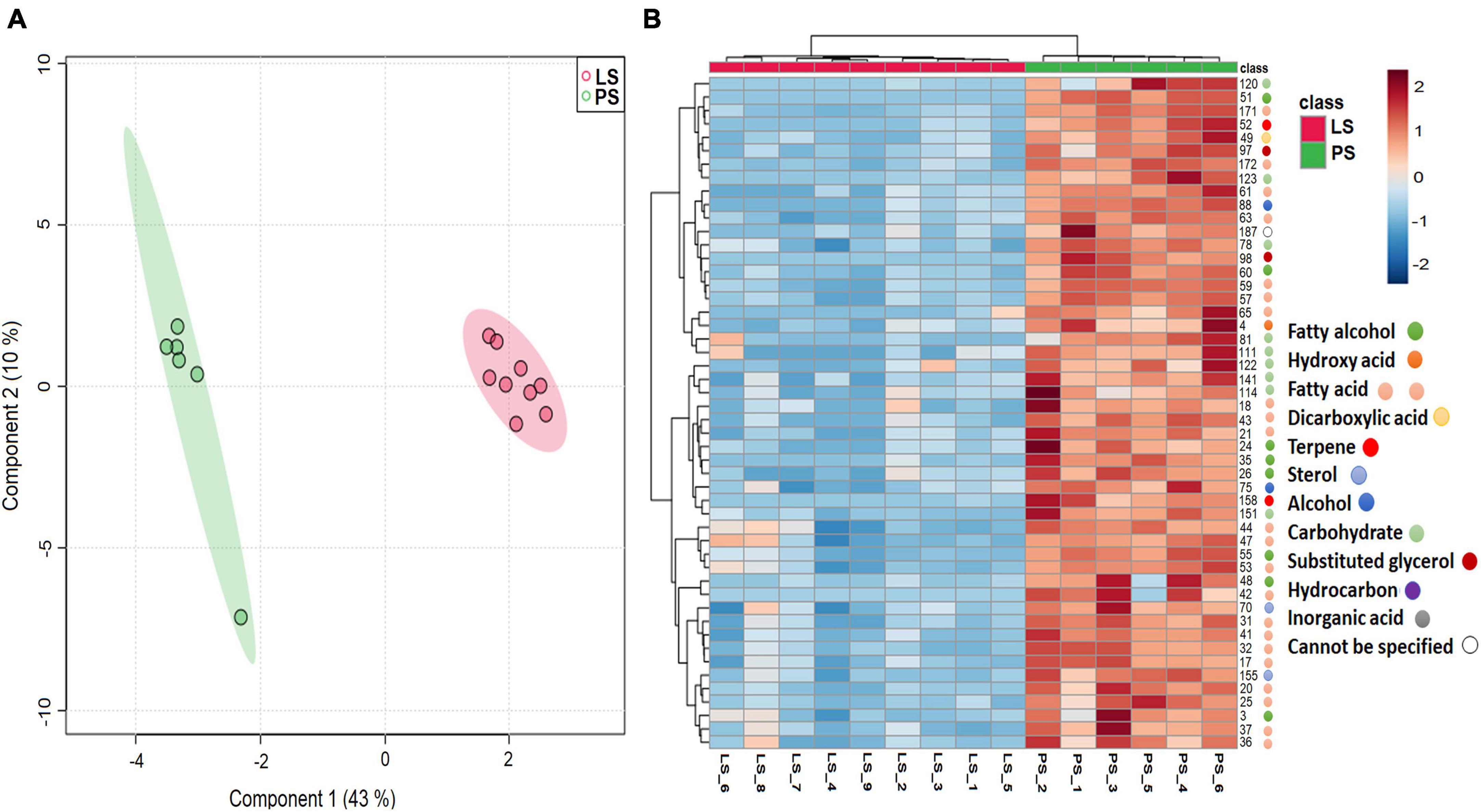
Figure 1. Soil Metabolite Profile. (A) Sparse partial least square discriminant analysis (sPLS-DA) plot representing the differences in the metabolite profiles in soil samples from two different sites, Lipova site (LS) with nine soil replicates and Prenet site (PS) with six replicates (B) Heatmap showing 50 most significant metabolites between the two soil samples (LS, PS) in replicates based on T-test and ANOVA.
3.3. Phospholipid fatty acids (PLFAs) analysis
The phospholipid fatty acids (PLFAs) analysis documents the differences in the microbial cell membrane composition to determine the presence of functionally active microbial communities in the soil (Cavigelli et al., 1995; Torsvik and Øvreås, 2002). Pernet (P) soil (71.7 ± 1.74 μg PLFA g–1 soil, p < 0.05) showed significantly higher viable microbial biomass compared to Lipova soil (22.7 ± 2.01 μg PLFA g–1 soil) (Table 2), indicating a high abundance of functionally active microbial communities in the soil with relatively higher amounts of TOC and TN. Although the bacterial (59.5 ± 1.34 μg PLFA g–1 soil, p < 0.05) and fungal (1.1 ± 0.09 μg PLFA g–1 soil, p < 0.05) biomass was higher in P soil compared to L soil, their ratio, i.e., bacteria: fungi ratio did not differ significantly between the two sites (P- site- 51.5 ± 3.77 μg PLFA g–1 soil, L-site- 57.3 ± 4.21 μg PLFA g–1 soil; p > 0.05). The PLFA estimates for gram-positive (7.83 ± 0.32 μg PLFA g–1 soil, p < 0.05), gram-negative bacteria (37.8 ± 1.08 μg PLFA g–1 soil, p < 0.05), and actinobacteria (13.4 ± 0.28 μg PLFA g–1 soil, p < 0.05) were significantly higher in P soil (Table 2).
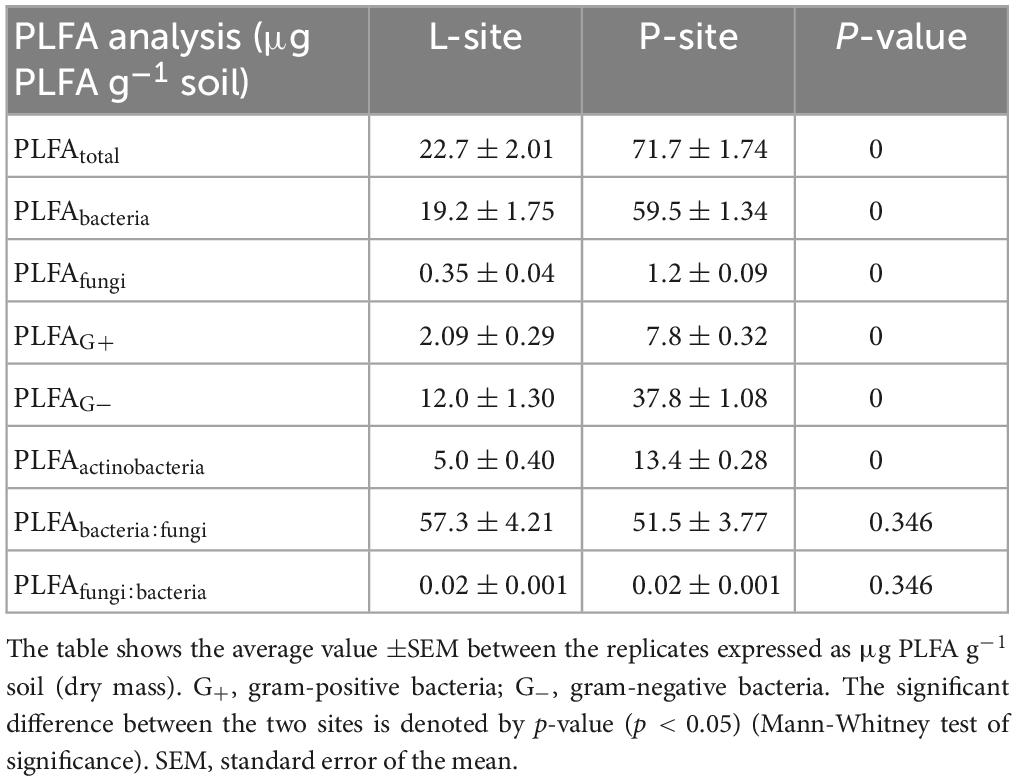
Table 2. Phospholipid fatty acid Analysis (PLFA) in two seed orchards, Lipova (L-site) and Prenet (P-site).
3.4. Soil microbial community composition and diversity
3.4.1. Quality control
The soil microbial community structure was characterized using Illumina paired-end amplicon sequencing targeting the bacterial 16S and the fungal ITS region. The soil samples from two sites (Lipova and Prenet) yielded 2,026,594 reads representing bacterial diversity, while 1,932,039 fungal reads were obtained. The initial quality check was performed with a set threshold of Phred Quality score >30. The reads with a Quality score <30 were discarded, and the clean reads obtained per soil samples for bacterial 16S and fungal ITS were further processed using bioinformatic data analysis pipelines (Supplementary Excel 2, 3).
3.4.2. Microbial communities in soil
The rarefaction curves and Good’s coverage index (>99%) represented the comprehensiveness of the sampling, indicating that the whole microbial diversity present in the soil samples was captured by sequencing (Supplementary Figure 2 and Table 3).
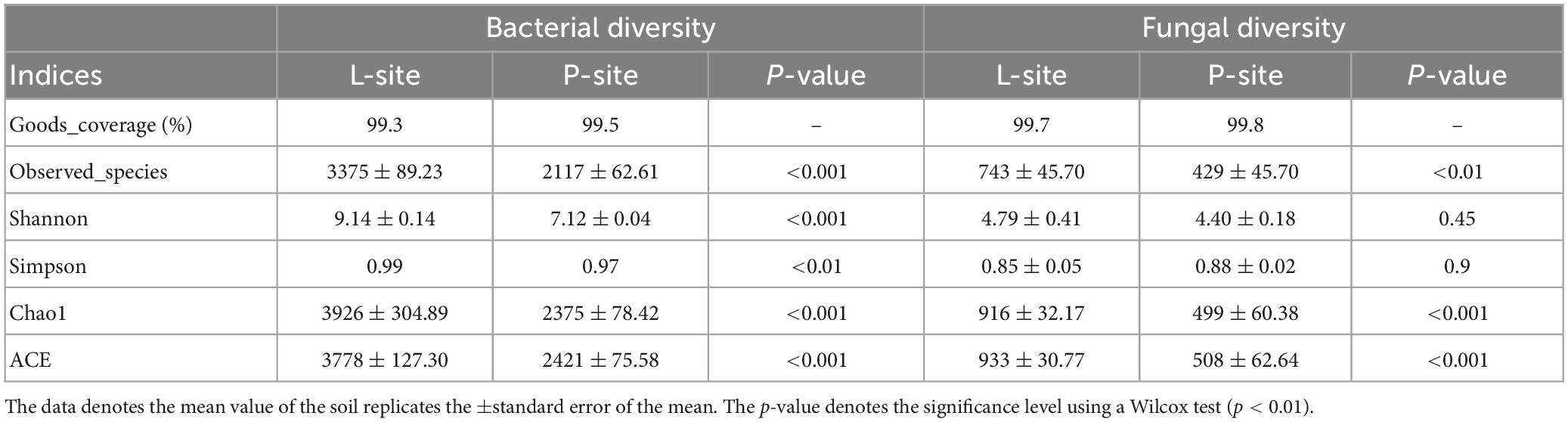
Table 3. Alpha diversity indices representing bacterial and fungal communities between two seed orchards, Lipova (L-site) and Prenet (P-site).
3.4.2.1. OTU (operational taxonomic unit) abundance
The Illumina sequencing of soil samples from two different sites resulted in a total of 6,389 bacterial and 2,288 fungal OTU clusters with a similarity threshold of 97% (Supplementary Excel 4, 5). Among the total OTUs detected in this study, 2,426 unique bacterial OTUs were present in Lipova soil, while only 382 unique OTUs were detected in Prenet soil (Figure 2A and Supplementary Excel 6). The top 10 bacterial order detected in the soil samples include Rhizobiales, Acidobacteriales, Gaiellales, Chthoniobacterales, Solirubrobacterales, Rhodospirillales, Solibacterales, Ktedonobacterales, Frankiales, Xanthomonadales (Figure 2B and Supplementary Excel 4). P soil showed a high relative abundance of Rhizobiales (36.2%), Acidobacteriales (10.9%), Chthoniobacterales (7.3%), Rhodospirillales (6.8%), Ktedonobacterales (4.2%), Frankiales (4.1%) while L soil documented a prevalence of Gaiellales (6.6%), Solirubrobacterales (5.3%), Solibacterales (4.9%), and Xanthomonadales (3.7%) (Figure 2B and Supplementary Excel 4).
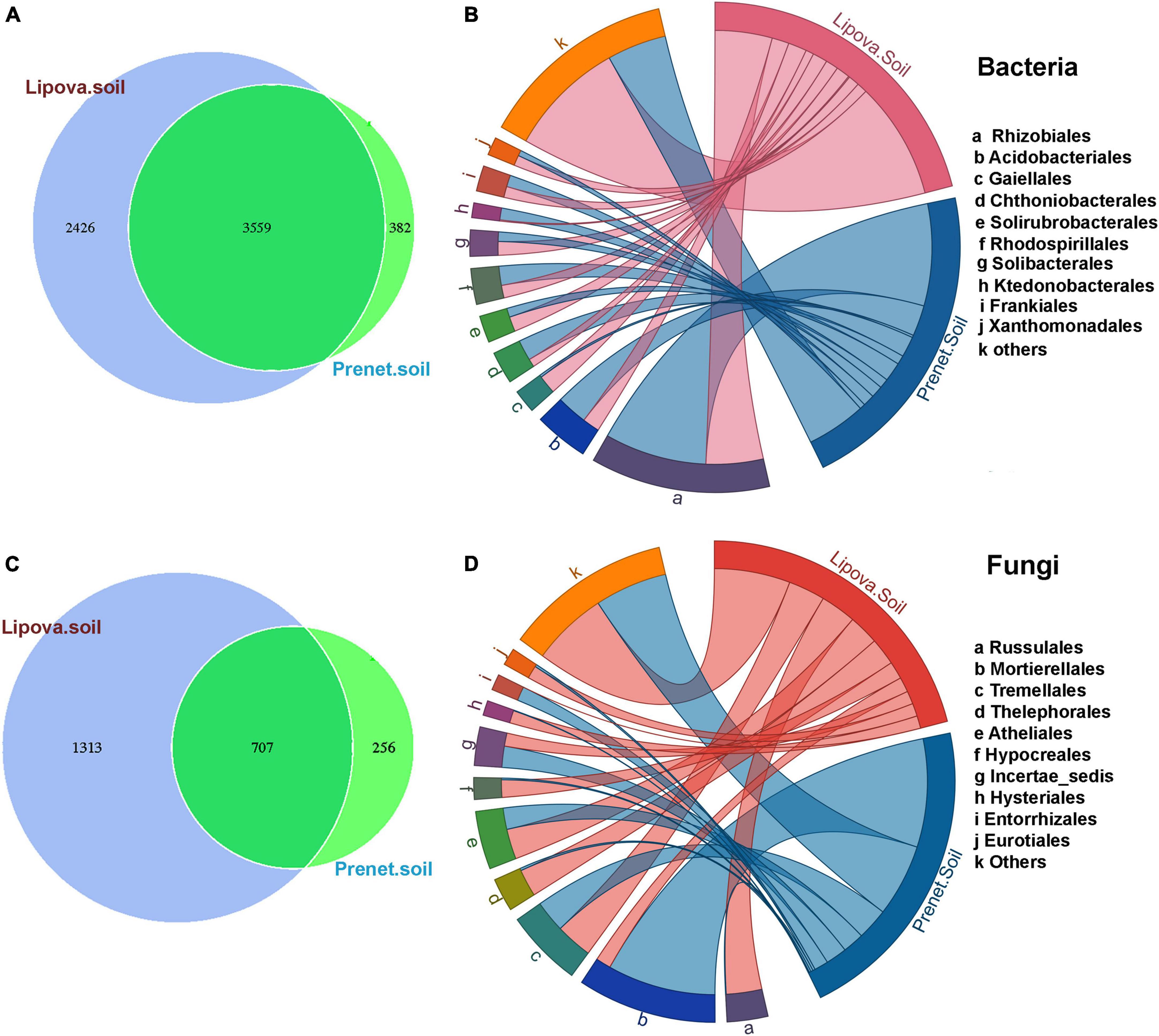
Figure 2. (A) Venn diagram showing the distribution of unique and common bacterial OTUs shared between two different soil samples (Lipova and Prenet soil). (B) Relative abundance of the top 10 bacterial orders observed in Lipova and Prenet soil. “Others” denote the relative abundance of the rest of the bacterial orders present in the soil. (C) Venn diagram showing the distribution of unique and common fungal OTUs shared between the two different soils. (D) Relative abundance of the top 10 fungal orders observed in Lipova and Prenet soil. “Others” denote the relative abundance of the rest of the fungal orders present in the soil.
Similarly, a high number of unique fungal OTUs (1313) were observed in Lipova soil compared to Prenet soil (256 OTUs) (Figure 2C and Supplementary Excel 7). Among the top 10 fungal orders observed in the soil samples, Mortierellales (39.2%), Incertae-sedis-Leotiomycetes (7.7%), and Entorrhizales (5.1%) were dominant in P soil. However, Russulales (12.9%), Tremellales (13.3%), Thelephorales (9.8%), Atheliales (10.9%), Hypocreales (6.3%), Hysteriales (4.5%) and Eurotiales (4.7%) were prevalent in L soil (Figure 2D and Supplementary Excel 5). Furthermore, comparing the biological replicates, Lipova (L) soil shared 1,511 core bacterial OTUs and 127 fungal OTUs (Supplementary Figures 3A, C and Supplementary Excel 8, 9). While the Prenet soil documented a consortium of 944 bacterial and 160 fungal core OTUs shared among the soil replicates (Supplementary Figures 3B, D and Supplementary Excel 10, 11).
The soil microbial communities were assigned to 41 bacterial and six fungal phyla. The Graphlan representation of the bacterial communities in Lipova soil showed the predominance of five phyla such as Proteobacteria, Firmicutes, Actinobacteria, Acidobacteria, and Bacteroidetes. However, apart from Proteobacteria, Firmicutes, Acidobacteria, and Actinobacteria, the Prenet soil documented the prevalence of Chloroflexi and Verrucomicrobia (Supplementary Figure 4). Similarly, the OTU tree representation using Graphlan showed the dominance of three fungal phyla, namely, Ascomycota, Basidiomycota, and Zygomycota, in both Lipova (L) and Prenet (P) soils (Supplementary Figure 5).
The evolutionary tree illustrated the relative abundance of the top 100 bacterial and fungal genera observed in the two soils (Supplementary Figure 6). The heatmap represents the relative abundance of 35 predominant bacterial and fungal genera observed in Lipova (L) and Prenet (P) forest soils (Figure 3).
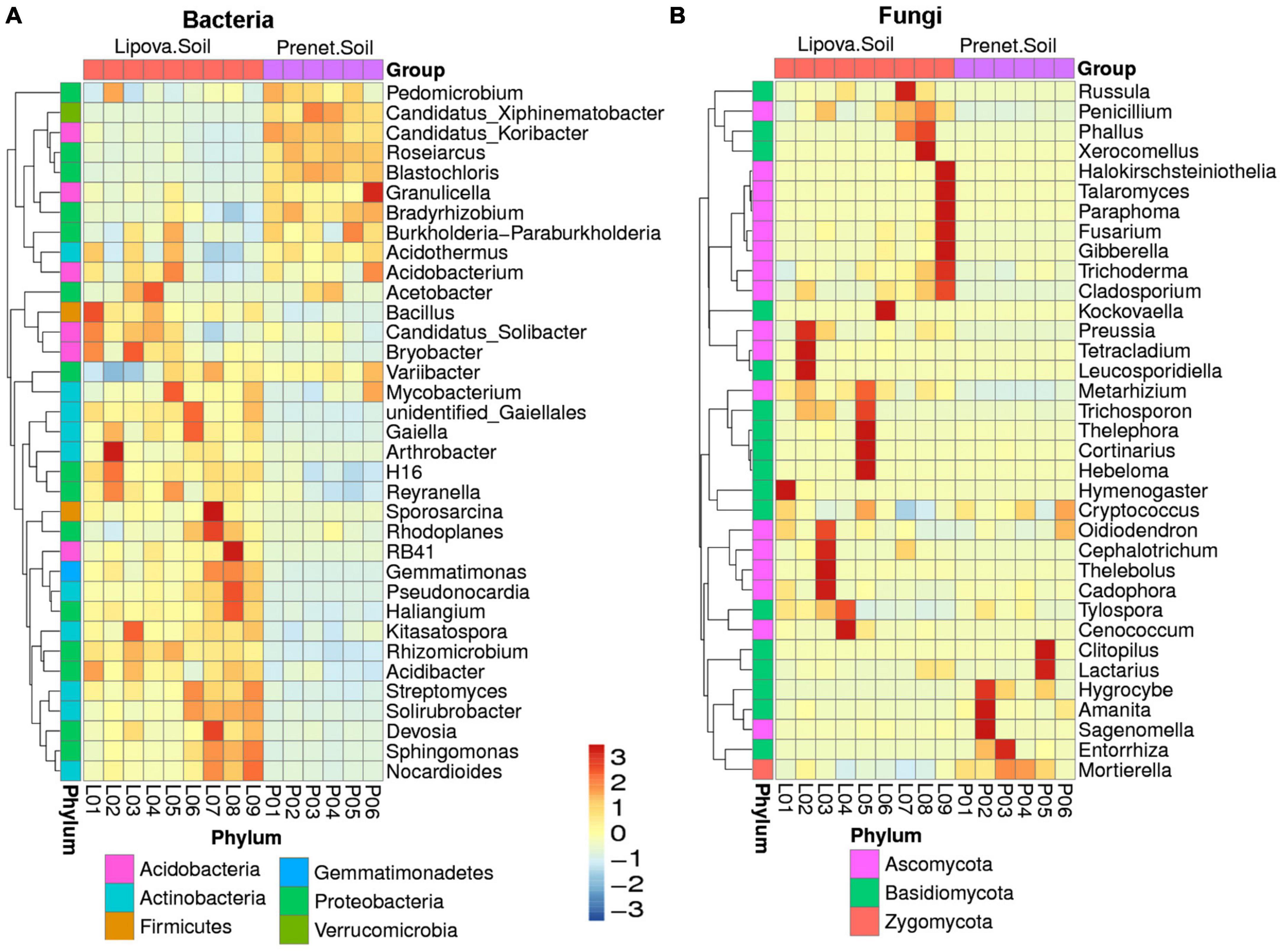
Figure 3. Heatmap illustrating (A) the relative abundance of 35 predominant bacterial genera in Lipova (L) and Prenet (P) soil samples with six replicates. (B) The relative abundance of 35 prevalent fungal genera in the soil samples with nine replicates. The color gradient indicates the relative OTU abundance for each soil sample, where the darker color denotes higher abundance, and the light colour represents low abundance.
3.4.2.2. Alpha diversity (α)
The alpha diversity indices represented the soil microbial diversity (richness and evenness) and community richness (Table 3). Bacterial diversity indicated by Shannon and Simpson index showed significantly higher diversity in L soil (Shannon index- 9.14; Simpson index- 0.99) compared to P soil (Shannon index- 7.12; Simpson index- 0.97) (Wilcox test, p < 0.01). The soil bacterial community richness showed a similar trend with high bacterial richness in L soil (ACE – 3778.06; Chao1- 3926.54) compared to P soil (ACE – 2421.56; Chao1- 2375.46) (Wilcox test, p < 0.01). Interestingly, the fungal diversity did not show any significant variation between the Lipova (L) (Shannon index- 4.79; Simpson index- 0.85) and Prenet (P) soil (Shannon index- 4.40; Simpson index- 0.88). However, the fungal community richness was considerably different between the two soils, where L soil documented high fungal richness (ACE- 933.28; Chao1- 916.10) (Wilcox test, p < 0.01) (Table 3).
3.4.2.3. Beta diversity (β)
The overall microbial diversity represented by the beta diversity based on Unweighted and Weighted UniFrac distances showed significant differences between the soil samples (Figure 4 and Supplementary Figure 7). The UPGMA hierarchical clustering illustrated the similarity among the different samples and grouped the soil samples in two distinct clusters suggesting the substantial difference in the soil microbial communities from two different sites (Figures 4B, D and Supplementary Figure 7). However, the UPGMA clustering of fungal communities based on weighted UniFrac clustered the biological replicates from Lipova soil into two clades, indicating soil heterogeneity (Supplementary Figure 7). Furthermore, the Non-Metric Multidimensional Scaling (NMDS) analysis documented the differences in soil microbiome from the two sites. The soil samples collected from two sites showed significant variation in their bacterial community structure, representing two distinct clusters (Figure 5A). Similarly, the fungal diversity between the two sites was substantially different (Figure 5B), suggesting the impact of site-specific environmental factors. However, such observation depicting the environmental influence on soil microbiota needs further validation.
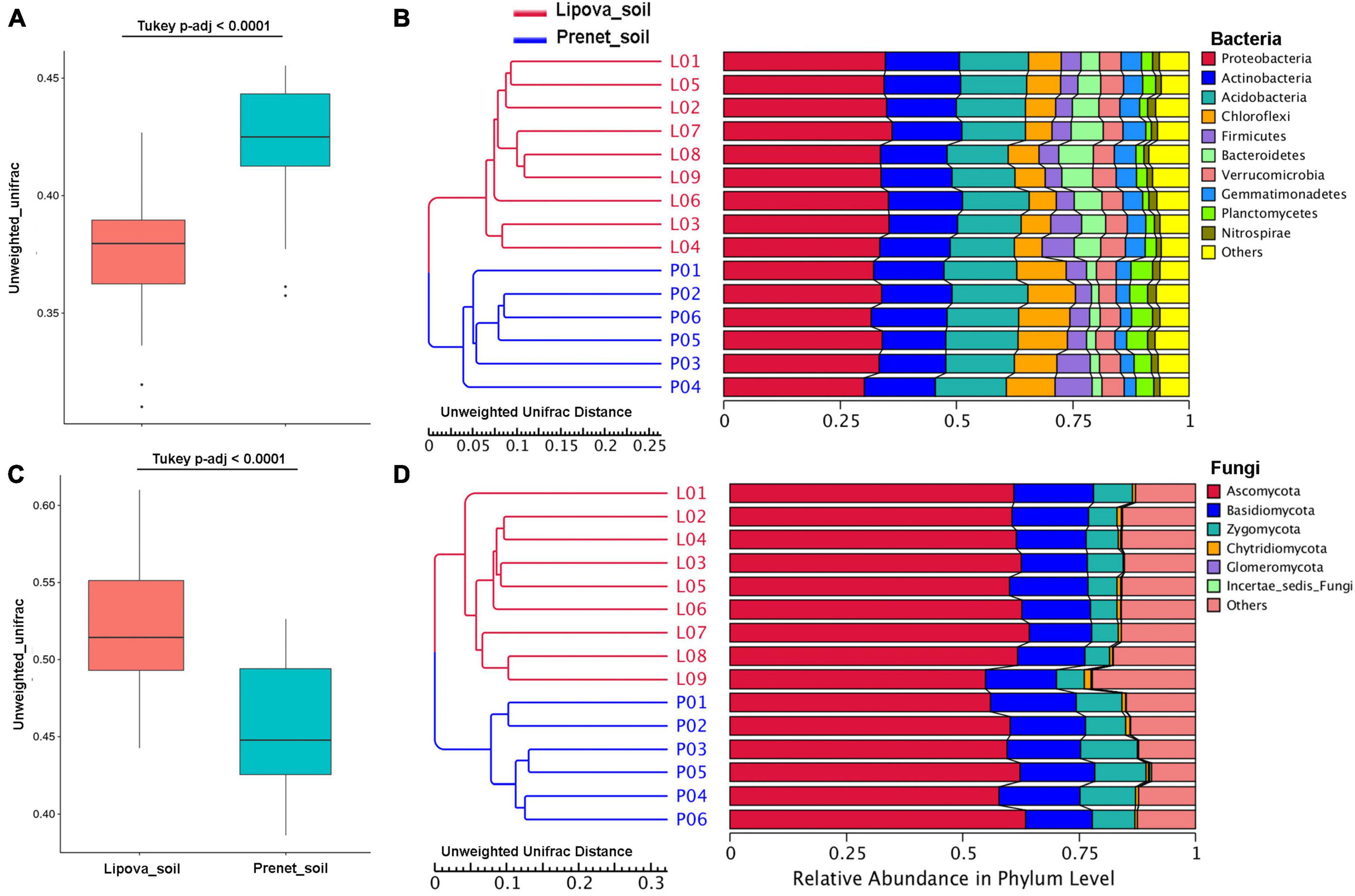
Figure 4. (A) Box plot based on the Unweighted Unifrac distance representing the variation in the bacterial communities present in soil samples from Lipova and Prenet. (B) Unweighted pair group method with arithmetic mean (UPGMA) tree clustering based on Unweighted Unifrac distance showing differences in the bacterial communities between the two soil samples in replicates. The relative abundance of the bacterial phyla is displayed along with the UPGMA tree. (C) Box plot based on the Unweighted Unifrac distance shows the difference in the fungal communities in Lipova and Prenet soils. (D) UPGMA tree based on Unweighted Unifrac distance illustrating the variation in the fungal communities between the two soil samples in replicates.
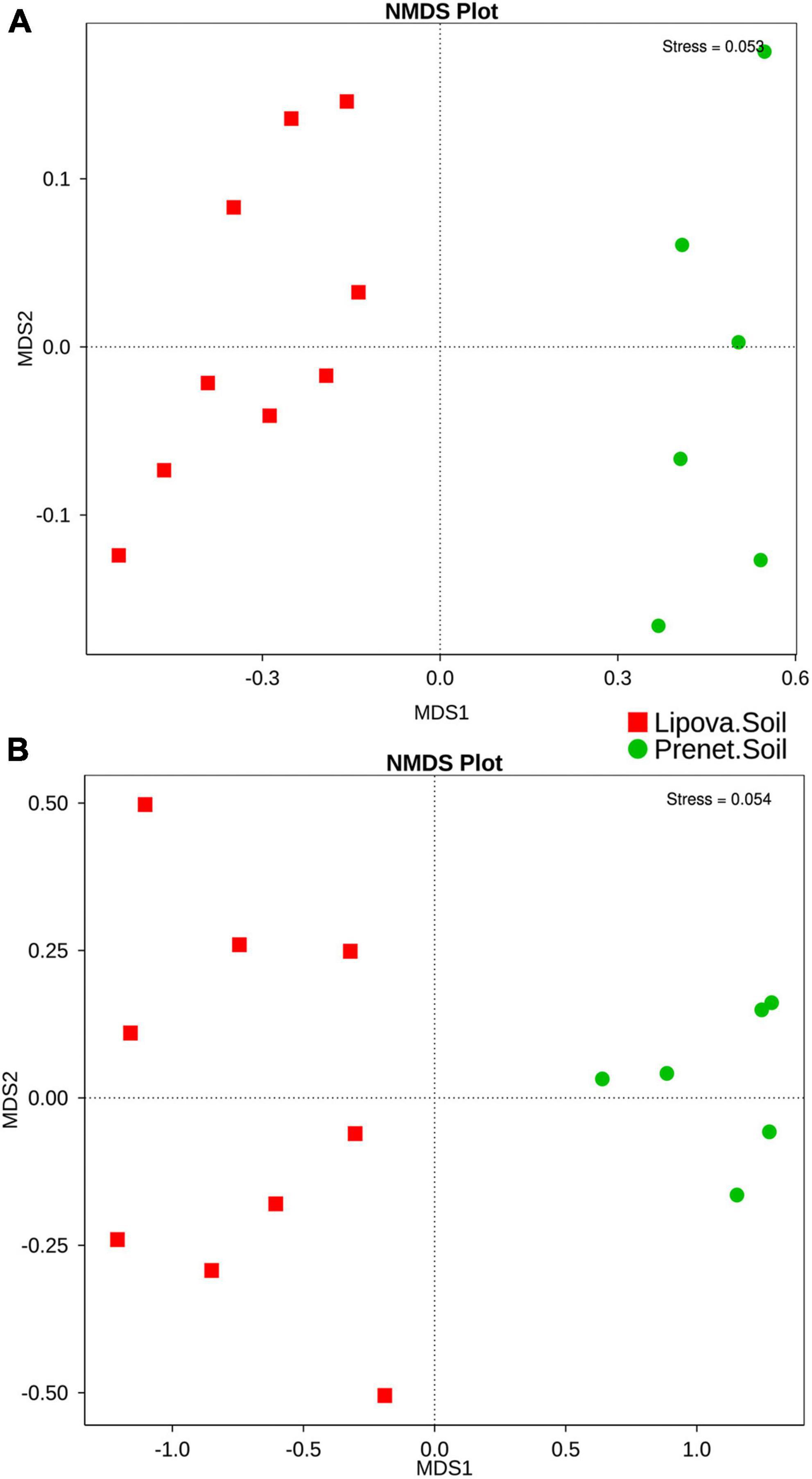
Figure 5. Non-metric multi-dimensional scaling analysis (NMDS) displaying (A) the difference in the bacterial communities in Lipova and Present soil. (B) The extent of variation in the fungal communities present in the soil samples. The data points in the same color represent soil samples from the same site. Different symbols designate the soil samples from different locations.
The differentially abundant microbes in the two different soil samples determined using T-test and Metastat analysis showed a predominance of different microbial communities between the sites (Figures 6, 7, Supplementary Figure 8). Furthermore, Analysis of Similarity (ANOSIM) determined significant variation in the microbial community structure between the two soils compared to the differences within the biological replicates (Supplementary Table 1). Likewise, MRPP analysis showed a substantial difference in microbial communities present in the two soils (Supplementary Table 2). Other statistical analyses, such as ADONIS and AMOVA, documented similar results (Supplementary Tables 3, 4). The study also revealed the soil microbial biomarkers that are significantly abundant and consistently present. The Linear discriminant analysis effect size (LEfSe) determined the different bacterial and fungal biomarkers present in the samples soil with a LDA score [log10] > 4 (Figure 8 and Supplementary Figure 9). The differentially abundant bacterial biomarkers in Prenet soil include members from Acidobacteria (class- subgroup 2; order- Acidobacteriales; family- Acidobacteriaceae_subgroup 1), Proteobacteria (class- Alphaproteobacteria; order- Rhizobiales, Rhodospirillales; family- Bradyrhizobiaceae, Roseiarcaceae, Xanthobacteraceae), Chloroflexi (class- Ktedonobacteria; order- Ktedonobacterales; family- HSB_OF53_F07), Verrucomicrobia (class- Spartobacteria; order- Chthonlobacterales; family- Xiphinematobacteraceae). However, Lipova soil was documented by the presence of bacterial biomarkers belonging to Bacteroidetes (class- Sphingobacteriia; order- Sphingobacteriales), Actinobacteria (class, Thermoleophilla; order- Gaiellales; Soilrubrobacterales), Acidobacteria (class- subgroup 6), Proteobacteria (class- Betaproteobacteria, Gammaproteobacteria, Deltaproteobacteria) (Figure 8A). Similarly, the differentially abundant fungal biomarkers in Prenet soil belonged to Zygomycota members (class- Incertae_sedis_Zygomycota; order- Mortierellales; family- Mortierellaceae) and Basidiomycota (class- Entorrhizommycetes). In contrast, the biomarkers in Lipova soil consisted of fungal species belonging to Dothideomycetes, Eurotiomycetes (Herpotrichlellaceae, Chaetothyriales, Trichocomaceae, Eurotiales), Hypocreales (Nectriaceae), Russulales (Russulaceae) (Figure 8B).
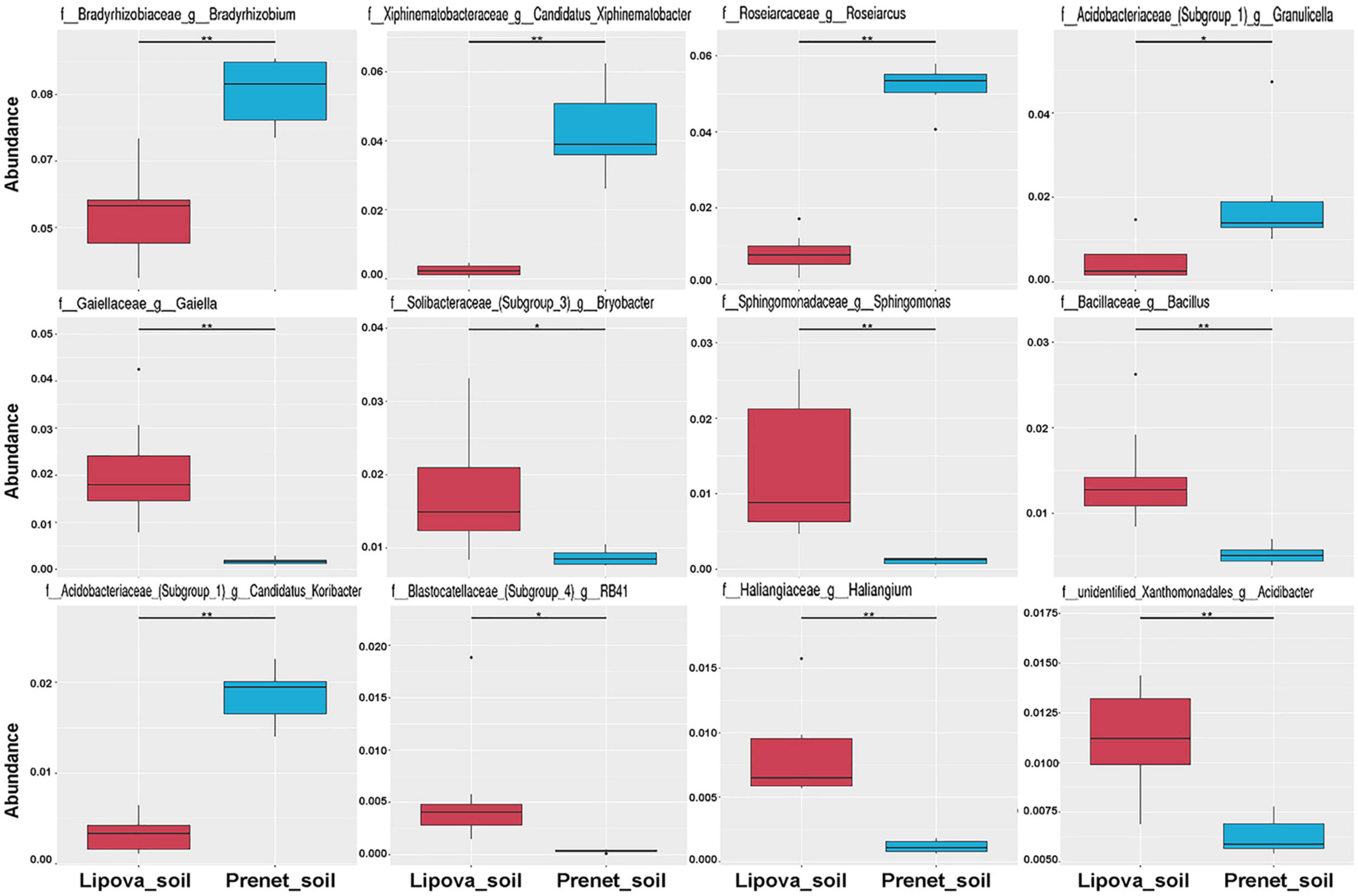
Figure 6. Metastats analysis shows significantly abundant bacterial species in Lipova and Prenet soil. The significant differences in the relative abundance are evaluated by the FDR test where the “*” represents significant variation at q value <0.05 while “**” denotes high significance at q value <0.01.
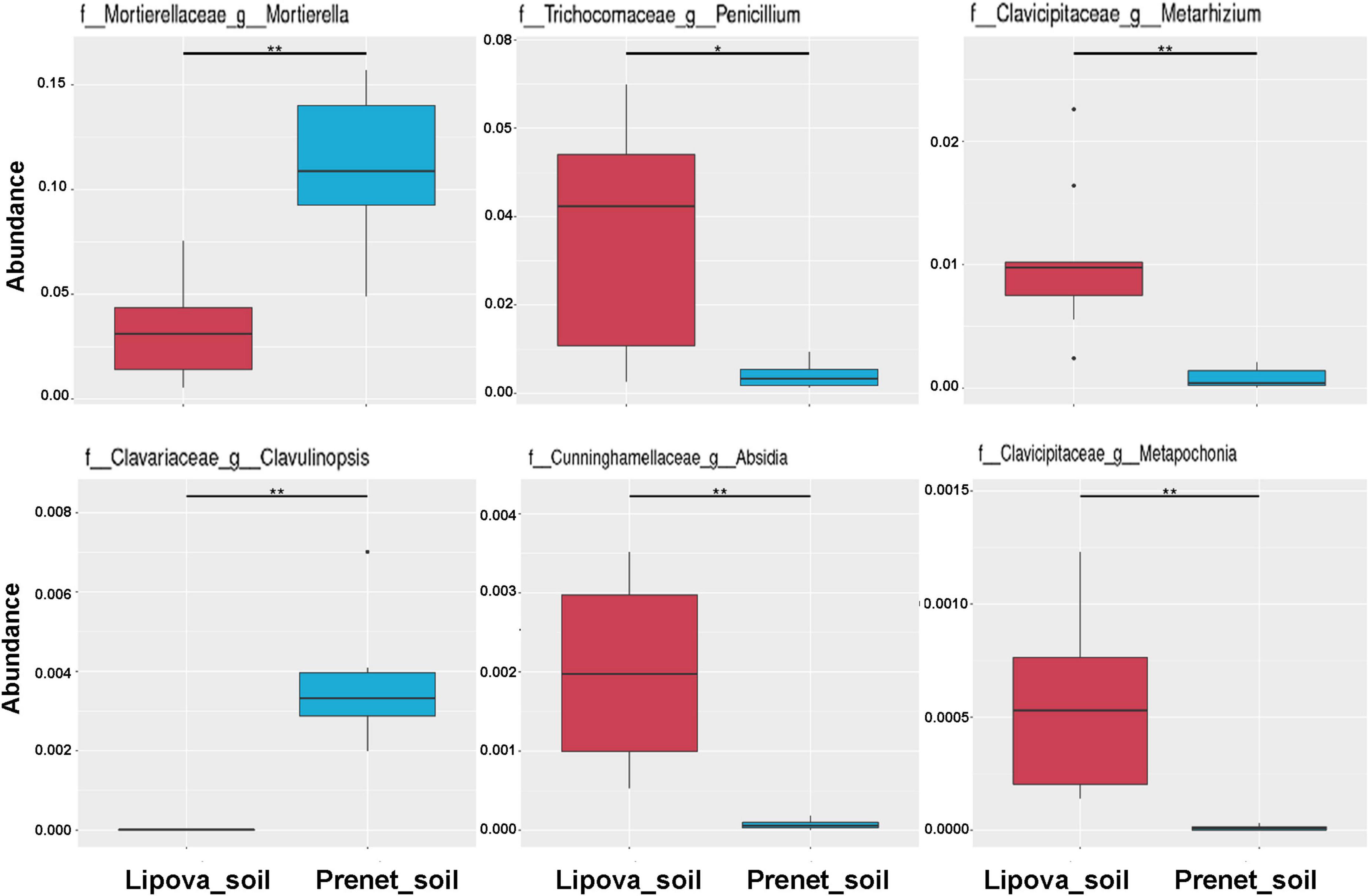
Figure 7. Metastats analysis represents significantly abundant fungal species in Lipova and Prenet soil. The significant variation in the relative abundance is estimated by the FDR test where the “*” represents a significant difference at q value <0.05 while “**” denotes high significance at q value <0.01.
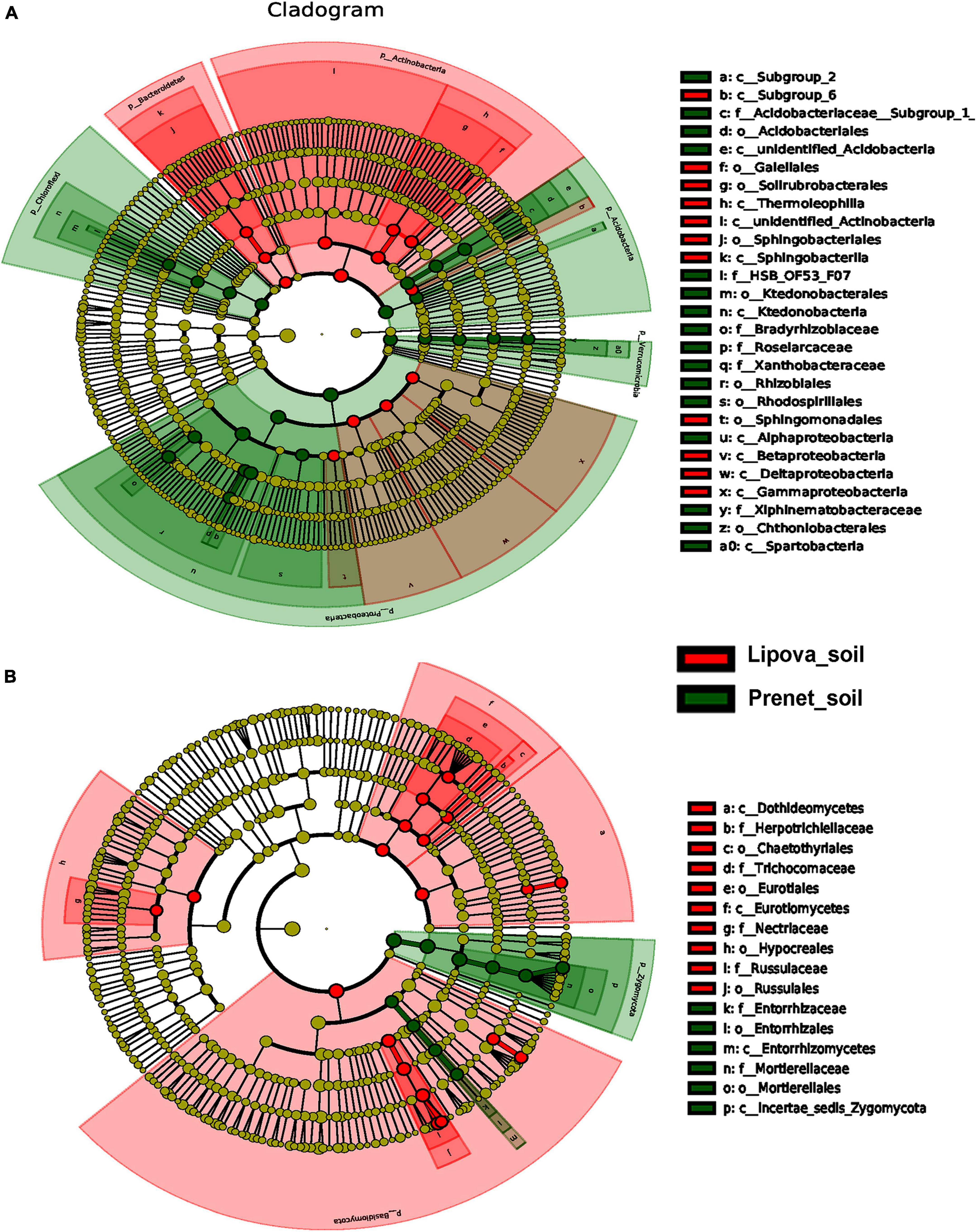
Figure 8. LEfSe [linear discriminant analysis (LDA) Effect Size] analysis indicating the differentially represented microbial biomarkers in Lipova and Prenet soil. (A) The cladogram illustrates the presence of bacterial communities that are significantly different between the two soil samples. (B) The cladogram represents the soil’s fungal biomarkers. The circles radiating from inside to outside denote the taxonomic level from phylum to genus. Each circle represents a distinct taxon at the corresponding taxonomic level. The size of each circle is proportional to the relative abundance of each taxon. Bacterial and fungal biomarkers with significant differences are colored according to the color of the corresponding soil samples, whereas yellowish-green circles resemble non-significant species. Red and green nodes denote that these species contribute highly to the group. The letters above the circles describe the different biomarkers.
3.4.2.4. Functional composition
PICRUSt analysis based on the relative abundance of the bacterial 16S gene sequences suggests the putative functional profile of the soil bacterial communities where each OTU is related to a function (Figure 9). The PCA plot based on the PICRUSt data revealed that the predicted functional gene composition between the two soil samples was different and clustered in two distinct groups (Figure 9C). The predicted gene functions include amino acid metabolism, lipid metabolism, terpenoids and polyketides, carbohydrate metabolism, membrane transport, DNA replication and repair, nucleotide metabolism, xenobiotics biodegradation, and metabolism of cofactors and vitamins were higher in L-site. In comparison, P-site showed a high abundance of putative functions mapped to signal transduction, genetic information processing, biosynthesis of secondary metabolites, glycan metabolism, energy metabolism, and transcription (Figure 9A). However, comparing the most abundant gene functions (top 10) in the soil samples did not significantly differ. The top 10 putative functions involve membrane transport, amino acid metabolism, carbohydrate metabolism, replication and repair, energy metabolism, xenobiotic metabolism, lipid metabolism, translation, and metabolism of cofactors and vitamins (Figure 9B).
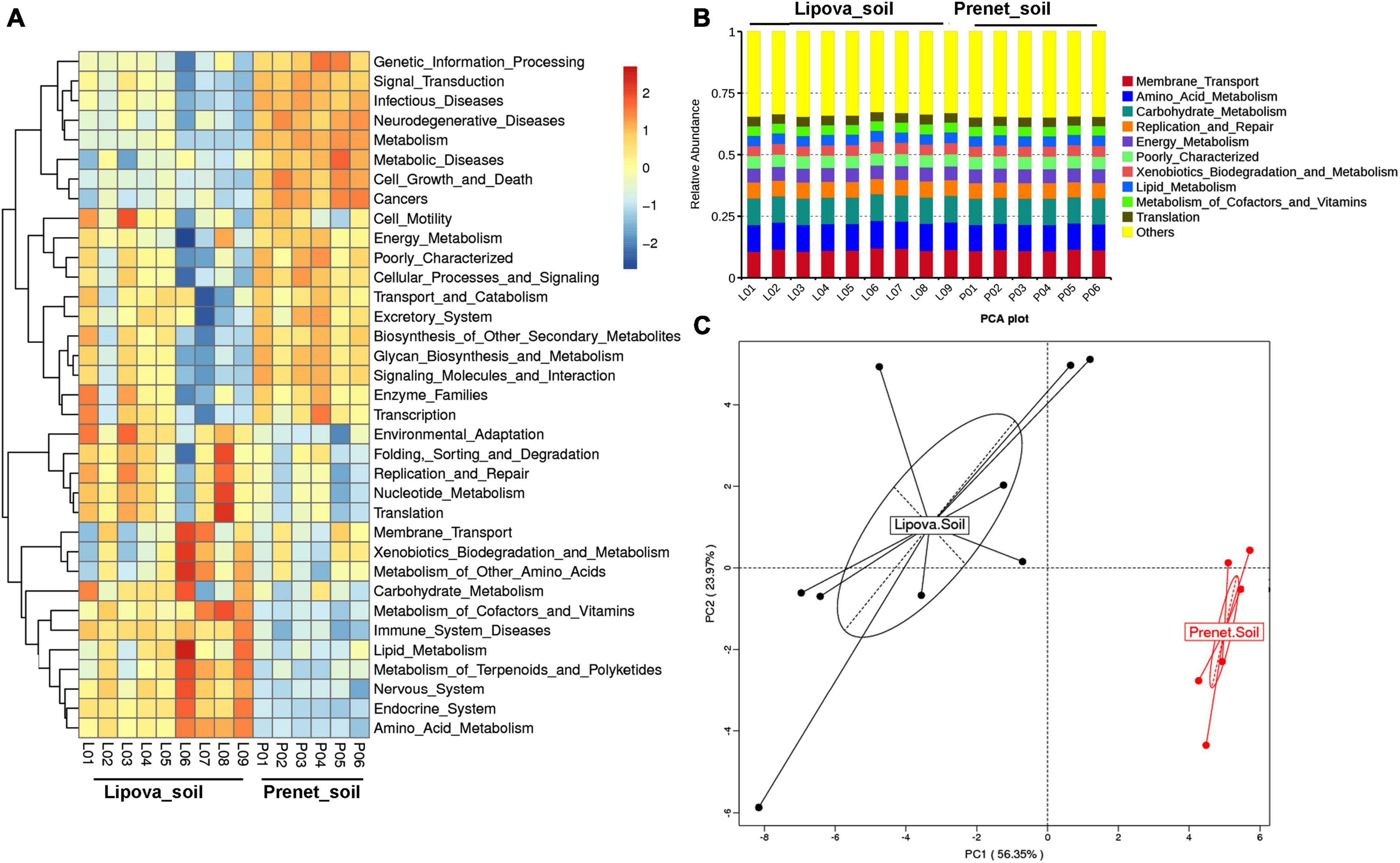
Figure 9. (A) Heatmap illustrating the functional profile predicted at level 2 KEGGs Orthologs using PICRUSt analysis represents the overall functional contribution of bacterial communities present in two soil samples with replicates. (B) The Barplot representing the relative OTU abundance contributing to the top 10 gene functions in soil shows no significant differences. “Others” represents the relative OTU abundance for the rest of the gene functions. (C) PCA plot shows differences in the predicted functional contribution of soil bacteriome in two sites (Lipova and Prenet) based on PICRUSt analysis.
3.5. Correlation between edaphic drivers and soil microbiota
The Spearman rank correlation analysis showed a significant relationship between the microbial species abundance and environmental factors such as pH (often acts as a key driver), conductivity, total organic carbon (TOC), and total nitrogen (TN) (p < 0.05). The pH, TOC, and TN substantially influence the abundance of bacterial communities compared to the fungal species (Figure 10). The bacterial genera such as Streptomyces, Kitasatospora, Devosia, Reyranella, Pseudonocardia, Gemmatimonas, Arthrobacter, Nocardioides, Solirubacter, Rhizomicrobium, Acidibacter, Haliangium, RB41, Sporosarcina, Bacillus, Sphingomonas, Bryobacter, and Gaiella were negatively correlated with TOC and TN. While Blastocholris, Pedomicrobium, Candidatus Koribacter, Granulicella, Roseiarcus, Candidatus Xiphinematobacter, and Bradyrhizobium showed a positive correlation (Figure 10A). On the contrary, soil pH showed a significant positive correlation with Streptomyces, Devosia, Pseudonocardia, Gemmatimonas, Nocardioides, Solirubacter, Haliangium, RB41, Sphingomonas, Gaiella. The bacterial genera such as Granulicella, Acidothermus, Roseiarcus, Candidatus Xiphinematobacter, and Bradyrhizobium were negatively correlated. Soil conductivity did not significantly influence the bacterial communities except for Variibacter and Candidatus Solibacter (Figure 10A). Furthermore, the mantel test analysis showed a significant correlation between the environmental variables (pH, TOC, TN, Conductivity) and the bacterial communities (r = 0.7497, p = 0.001).
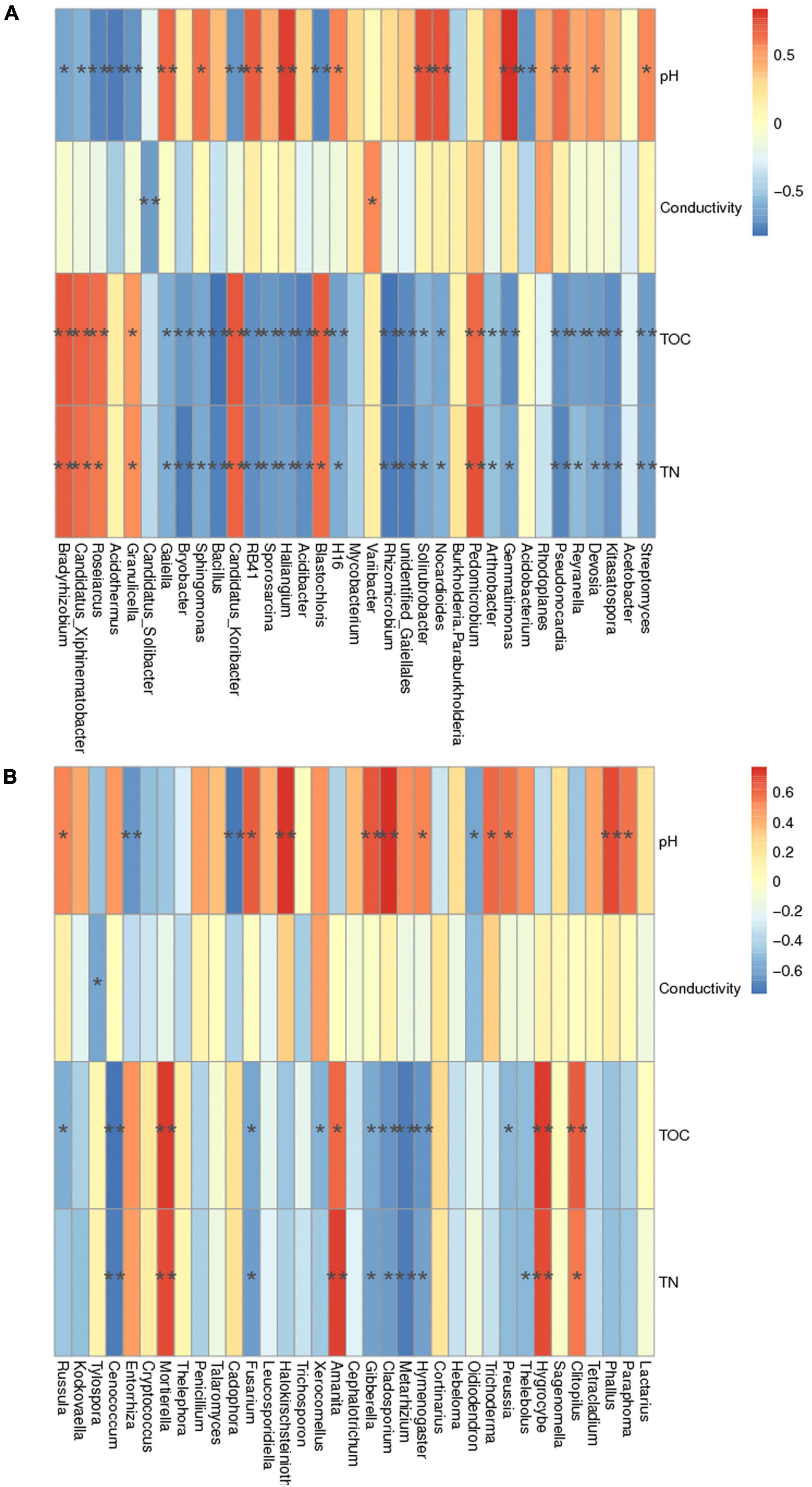
Figure 10. Heatmap illustrating the Spearman correlation between the environmental factors such as pH, Conductivity, Total organic carbon (TOC), Total nitrogen (TN) and (A) the bacterial species abundance (alpha diversity), (B) the fungal species abundance to obtain the correlation and significance between the two variables. The columns denote environmental factors, and the rows indicate the species. Colored tiles correspond to the Spearman rank correlation coefficient r value, that range from −1 to 1. r < 0 indicates a negative correlation; r > 0 is a positive correlation; ‘* denotes significance at p < 0.05.
Similarly, the abundance of fungal species such as Paraphoma, Phalas, Preussia, Trichoderma, Oidiodendron, Hymenogaster, Cladosporium, Gibberella, Halokirschsteiniothelia, Fusarium, Cadophora, Entorrhiza, Russula was significantly correlated with soil pH (p < 0.05) (Figure 10B). Furthermore, the TOC and TN content in soil showed a substantial effect on the abundance of Clitopilus, Hygrocybe, Thelebolus, Preussia, Hymenogaster, Metarhizium, Cladosporium, Gibberella, Xerocomellus, Amanita, Fusarium, Mortierella, Russula, and Cenococcum (Figure 10B). The fungal genus, Tylospora was only influenced by soil conductivity. Like bacterial communities, the mantel test analysis also demonstrated a significant correlation between the soil fungal abundance and the environmental variables (pH, TOC, TN, Conductivity) (r = 0.4115, p = 0.002) in two different seed orchards.
Additionally, the Canonical correspondence analysis (CCA) revealed the species-environment relationship illustrating four parameters, including soil pH, conductivity, total organic carbon (TOC), and total nitrogen (TN), that influence the development of specific microbial communities (Figure 11). The results indicate 91% of the bacterial community variance that could be explained by the mentioned edaphic factors (Figure 11A). The Lipova and Prenet soils were clustered in separate groups. Our data suggest that the first canonical axis (CCA1) was positively correlated with TOC and TN, whereas the second canonical axis (CCA2) was negatively correlated with soil pH and conductivity. Similarly, the soil parameters contributed to 64.87% of fungal community variation (Figure 11B). The Prenet soil was grouped separately, but Lipova soil was scattered. CCA1 axis was positively correlated with soil pH and conductivity, and the CCA2 was negatively correlated with TOC and TN (Figure 11). Furthermore, soil pH, TOC, and TN, represented by longer arrows, were the major edaphic variables that played a critical role in shaping the soil microbial community structure (Figure 11).
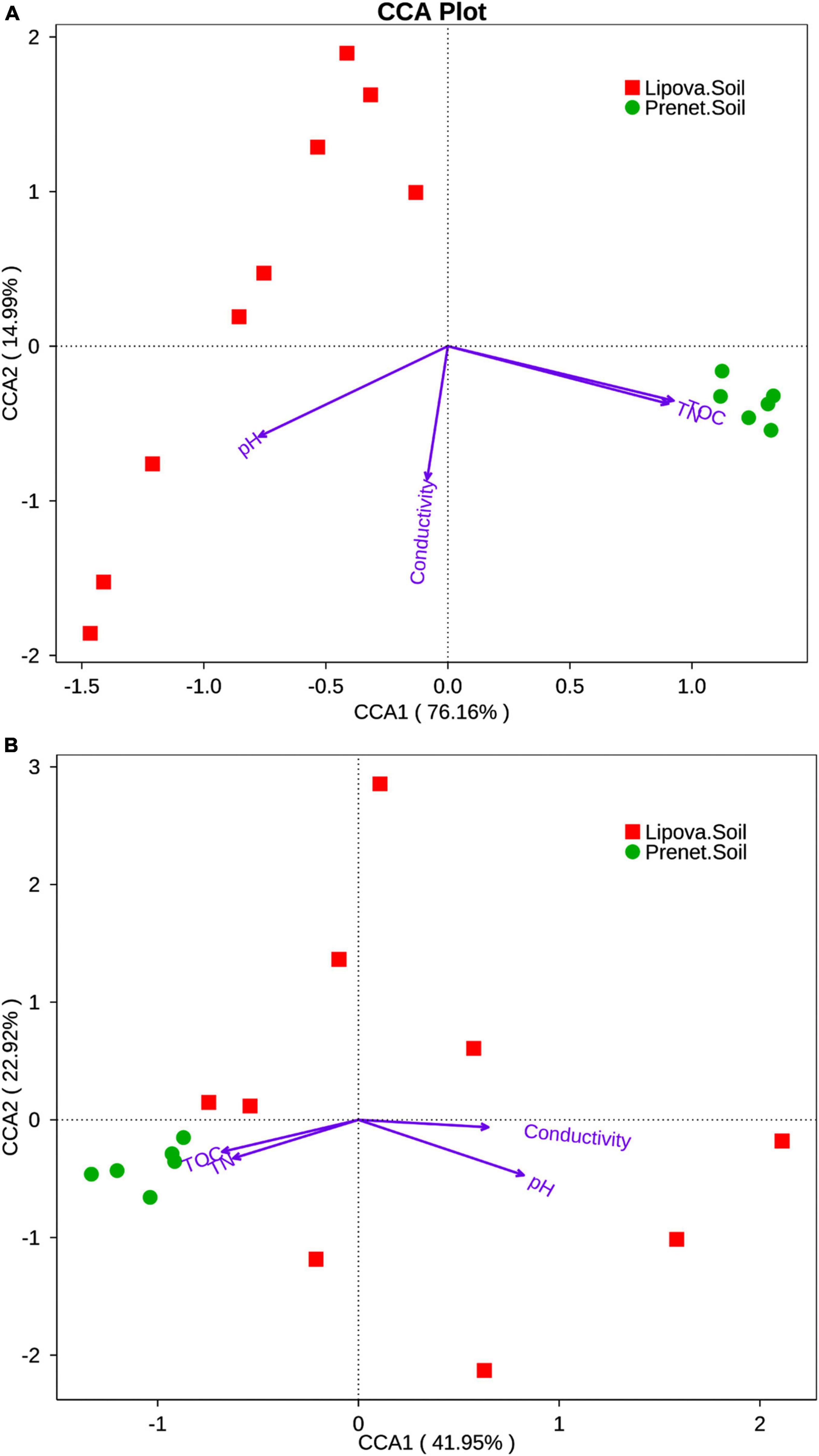
Figure 11. Canonical correspondence analysis (CCA) plot representing the relationship between the environmental factors such as pH, Conductivity, Total organic carbon (TOC), Total nitrogen (TN) and (A) the bacterial community distribution in soil samples (Lipova soil and Prenet soil) (B) the fungal distribution in Lipova and Prenet soil. The arrows indicate the association between environmental factors and microbial community distribution. The longer the arrows, the greater the association and vice versa. The angle between the arrow and ordination axes responds to the association between an environmental factor and the ordination axes. The less the angle, the greater the association and vice versa. The relationship between two environmental factors is positive when their included angle is acute and negative correlation when they are at an obtuse angle.
The Variation Partition Analysis (VPA) determines the relative contribution of edaphic factors (pH, conductivity. TOC, and TN) on soil microbial diversity (Figure 12). The data revealed that the soil physical characteristics (pH and conductivity) explained 6.5% of the total variation of the bacterial community structure. In comparison, the total organic carbon (TOC) and nitrogen (TN) contributed to 37.6% of the total variation of the soil bacterial communities, leaving 20.7% of the total variance unexplained (Figure 12A). Similarly, pH and conductivity contributed to 6.6% of the total variance of the soil fungal community, and TOC and TN explained 32.8% of the total variance of the fungal community structure. However, around 52% of the total fungal community variance was unaccounted (Figure 12B). The explained and unexplained variances in VPA were calculated as reported earlier (Legendre, 2008).
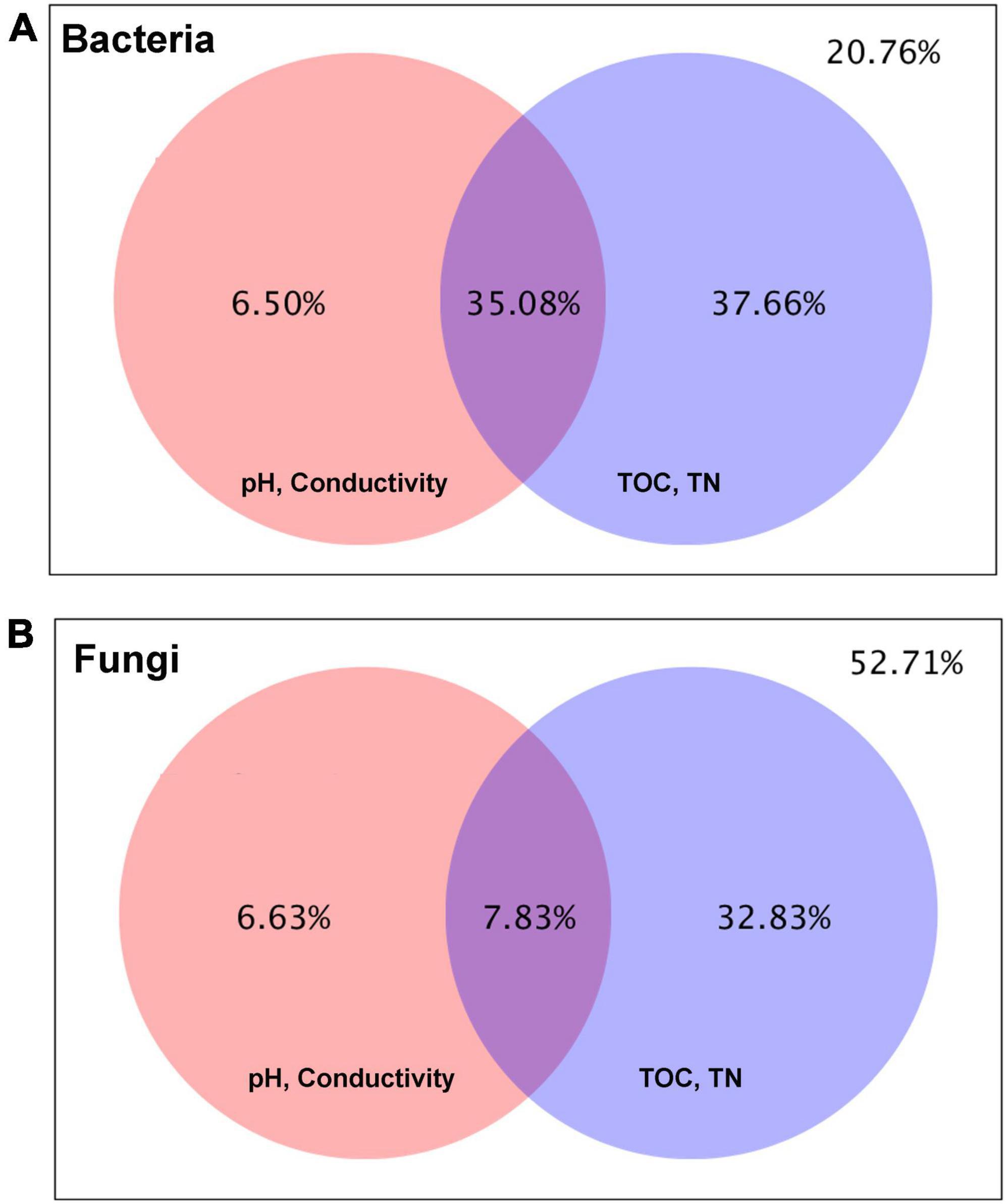
Figure 12. Variance partitioning canonical correspondence analysis (VPA) displaying the total variance of (A) the bacterial community, (B) the fungal community distribution into the respective contributions and covariations of each set of environmental variables. The outside value indicates the percentage of variance that cannot be explained.
4. Discussion
Anthropogenic disturbances and global climate change broadly impact the proper functioning of the forest ecosystem. Variation in precipitation amounts and patterns is one of the foremost effects of global climate change (Stocker, 2014). The alteration in the soil moisture content due to soil texture and precipitation directly or indirectly affects the ecosystem functions and processes (Sorensen et al., 2013; Patel et al., 2021). Soil moisture content is one of the main constraints influencing the soil microbial community structure-function (Li et al., 2016) and affects the nutrient availability in soil (Xu et al., 2014). In the present study, we explored the effect of precipitation and higher soil water content on various edaphic factors and the soil microbial community structure in two Norway spruce seed orchards. The average annual precipitation recorded over three decades is nearly double in Prenet (P) site compared to Lipova (L) site (Supplementary Figure 1). Interestingly, soil texture significantly influences the soil water-holding capacity and availability (Aina and Periaswamy, 1985; Vereecken et al., 1989). The low water content in L-site soil is correlated with the high sand percent in the L- site soil compared to the P- site (Li et al., 2009). The increase in clay and silt content in soil decreases the water permeability, thereby increasing the water-holding capacity (Wang et al., 2022). On the contrary, the water retention capacity decreases with the increased sand content in the soil. Nevertheless, our study demonstrates the relationship between various edaphic factors and the soil microbial community composition in two sites with significant differences in their precipitation rate.
4.1. Effect of precipitation on the soil factors
The present study documented substantial variation in the total organic carbon (TOC) and nitrogen (TN) content with the difference in the annual precipitation rate between the two sites. The higher amounts of TOC and TN measured in Prenet (P) soil were attributed to increased precipitation at this site (Borken and Matzner, 2009; Bell et al., 2014). Moreover, soil texture, more specifically the clay content in the soil, also plays a crucial role in the accumulation of the soil organic carbon (Franzluebbers et al., 1996; Dexter, 2004) and moisture content in the soil. Hence, higher clay and silt percent and high water content in P site soil influence the total organic carbon content compared to L site soil. Increasing soil moisture content is expected to increase litter turnover, thereby increasing soil C sequestration. Many studies reported that a decrease in water availability negatively impacts soil C sequestration (Burke et al., 1989; Zhou et al., 2002). However, such assumptions are recently questioned (Bowden et al., 2014; Fekete et al., 2016). Water availability promotes plant growth and increases biomass and litter production. Increasing litter turnover can enhance microbial activity, promoting decomposition and soil respiration and decreasing soil C sequestration (Fontaine et al., 2007; Kuzyakov, 2010) and vice versa (Fekete et al., 2014, 2017). For instance, studies reported reduced soil C accumulation in the forests receiving higher annual precipitation (Meier and Leuschner, 2010; Chen et al., 2016).
Nevertheless, the increase in water availability promotes soil microbial growth and impacts their activity (Liu et al., 2009), thereby enhancing soil carbon release (Huang et al., 2015). Due to global warming, changes in the precipitation pattern have decreased the soil moisture content (Zhou et al., 2011). Soil moisture is a crucial factor in controlling the organic carbon turnover in soil (Zhou et al., 2005). Therefore, it is vital to understand the effect of precipitation on the soil C dynamics. Studies showed that increased precipitation releases more dissolved organic carbon from the soil aggregates as accessible substrates resulting in increased microbial activity and higher CO2 emissions (Wu et al., 2011; Wang et al., 2021). Although precipitation change can control variations in soil pH at the global scale (Slessarev et al., 2016), our results showed a marginal difference in the soil pH between the two sites that are not far apart.
4.2. Effect of precipitation on soil metabolite profile and soil microbial activity
The soil moisture, pH, and organic matter content are crucial factors controlling the microbial community structure and carbon cycling in soil (Judd et al., 2006; Collins et al., 2014; Xu et al., 2020). Several studies reported a positive correlation between microbial diversity and soil organic matter across different ecosystems (Berthrong et al., 2013; Maestre et al., 2015). Soil pH also influences the metabolite profile in soil. For example, higher soil pH showed high citrate and low malate concentrations in soil and vice versa (Veneklaas et al., 2003). Similarly, precipitation change also influences microbial community structure (Barnard et al., 2013) by impacting the soil moisture and organic matter content (Maestre et al., 2016). Such a strong link between soil moisture, organic matter content, and the microbial community structure is critical in nutrient cycling (Elbert et al., 2012).
Soil microbes utilize the dissolved organic carbon in the soil, including root exudates, which also influence the soil microbial community structure (Swenson et al., 2015) and plant functions (Pétriacq et al., 2017). These soil metabolites are often used as biomarkers to reflect the responses of soil microbes to various conditions (Lankadurai et al., 2013; Jones et al., 2014). For instance, anaerobic soil disinfestation altered the soil metabolite profile and shifted the soil microbial communities (Johns et al., 2017). Our study documents distinct metabolite profiles in soil from two Norway spruce seed orchards with long-term precipitation differences over three decades. A distinct difference in the metabolite composition resulted in the clustering of soil samples according to location (Figure 1). Johns et al. (2017) suggested that soil metabolomics links organic/inorganic compounds and microbes in the soil. Hence, our results indicate varied microbial functions between the soils collected from Prenet and Lipova.
Lipova (L) site received less annual precipitation and showed lower soil moisture content than the Prenet (P) site. Lower moisture content in soil affects the decomposition rates of the plant materials such as litterfall and roots (Martin et al., 2004; Ostertag et al., 2008). Such slow decomposition of the plant materials could be due to decreased microbial biomass and insufficient microbial activity. The PLFA analysis determining the spatial soil microbial community structure (Zelles, 1999) showed lower microbial biomass indicating a low abundance of functionally active microbial communities in the Lipova soil. On the contrary, increased precipitation in Prenet soil documented higher microbial biomass based on the total PLFA estimate. The soil water content significantly influenced the microbial biomass and its activity. Such observation corroborates previous studies where higher water availability in soil increased microbial biomass (Williams and Rice, 2007; Huang et al., 2015). Alternatively, water scarcity during drought often reduces the microbial PFLA (Hueso et al., 2012) due to decreased microbial growth by allotting more resources to avoid dehydration, reduced mobility, diffusion of solutes, and availability of nutrients (Harris, 1981; Kieft, 1987; Schimel et al., 2007). The PLFA content (total and the signature PLFAs) in soil was positively correlated with the TOC and TN. The availability of organic nutrients is a crucial factor influencing the soil microbial biomass (Wagai et al., 2011; De Vries et al., 2012).
Furthermore, the PLFA (fungi/bacteria) ratio indicates the change in soil microbial community structure (Zeglin et al., 2013). Although increased precipitation enhanced the microbial PLFA, i.e., bacterial and fungal PLFA content in P soil, it did not significantly influence their ratio (PLFA fungi/bacteria) between the two sites (Table 2). Similarly, the PLFA ratio between the gram-negative and gram-positive bacterial biomass is considered a stress indicator (Klamer and Bååth, 1998). The thicker cell wall of gram-positive bacteria makes them more resistant to water scarcity than gram-negative bacteria. However, our results showed no considerable influence of increased precipitation on the PLFA (G+/G-) ratio between the sites. Such observation might suggest that the soil microbes readily adapt to their environment and sustain a stable community structure (Huang et al., 2015; Yang et al., 2017).
One way the microbial communities in the soil contribute to nutrient cycling is by producing extracellular enzymes (Bowles et al., 2014). These extracellular enzymes facilitate the degradation, transformation, and mineralization of soil organic matter (Sinsabaugh, 2010; Xiao et al., 2018) and influence soil quality and ecosystem productivity (Sayer et al., 2013). Our data showed a significant increase in extracellular enzyme activities (EEAs) with increased microbial biomass under elevated precipitation. Precipitation alleviates microbial physiological stress and promotes microbial activity by increasing nutrient availability (Austin et al., 2004; Tiemann and Billings, 2011; Manzoni et al., 2012). Consequently, higher organic carbon and nitrogen content levels were positively correlated with the EEAs in soil. The selected extracellular enzymes in this study were involved in the decomposition of soil organic carbon (α -glucosidase, β-glucosidase, β-galactosidase, β-xylosidase, cellobiohydrolase), nitrogen (chitinase), and phosphorous (acid phosphatase) to their assimilable forms (Bell et al., 2013). Prenet soil with higher organic carbon content documented higher activity of these enzymes, consistent with the earlier studies (Sinsabaugh et al., 1991). Increased precipitation enhances the responses of soil microbial functional genes involved in biogeocycling (Li et al., 2017). Hence, precipitation is a critical factor that influences enzyme production and turnover that impacts the responses of soil enzymes and ecosystem productivity (Yang et al., 2017).
Microbes primarily produce extracellular enzymes in soil, but certain enzymes are limited to particular microbial taxa. For instance, lignocellulose degrading enzymes and chitinases are commonly produced by fungi (Baldrian and Valášková, 2008; Sinsabaugh et al., 2008). Fungi can process recalcitrant nutrient-limiting substrates, while bacteria readily decompose labile substrates (Xu et al., 2015; Treseder et al., 2016). Fungi secrete extracellular enzymes that can readily decompose complex plant organic matter and provide bacteria with the labile substrate (Romaní et al., 2006). However, the correlation between the microbial communities and the enzyme activities is underexplored.
In the present study, the soil microbial composition showed significant variation between the two sites, with higher bacterial and fungal diversity in L-site soil. In contrast, significantly higher soil extracellular enzyme activities (EEAs) and the total PLFA were observed in P-site soil, indicating the high abundance of metabolically active microbes that might play an essential role in organic matter recycling (Coolen and Overmann, 2000). Such observation could be correlated with the high water content in P-site soil, which positively impacts microbial activity and increases extracellular enzyme activities (Borowik and Wyszkowska, 2016).
4.3. Effect of precipitation on soil microbial community structure
The present study demonstrates the variation in soil microbial communities across two seed orchards receiving different precipitation amounts. Lipova site receiving less precipitation documented higher microbial diversity, low microbial biomass and soil organic carbon. The soil microbial diversity and biomass are the major drivers of ecosystem processes, such as nutrient cycling, organic matter decomposition, etc., (Crowther et al., 2016). Although several studies have shown the influence of biotic and abiotic factors in controlling soil microbial diversity and biomass (Tedersoo et al., 2014; Fierer, 2017; Delgado-Baquerizo et al., 2018), the correlation between the microbial diversity and biomass and the factors controlling them is still limited. One of the important abiotic factors influencing the retention of organic matter, microbial biomass and microbial activity is the soil texture (Bechtold and Naiman, 2006). The presence of finer soil particles (high clay and low sand percentages) constitutes more stable aggregates in soil and tends to accumulate higher amounts of organic C and total nitrogen contents, thereby strongly influencing nutrient availability (Raiesi, 2006). Additionally, soil texture significantly impacts the abundance of the microbial (bacteria and fungi) population. It was suggested that as the soil organic content increases, the abundance of dominant taxa is promoted, thereby increasing the microbial biomass (Bastida et al., 2021). However, such an increase in the microbial biomass reduces the diversity of the subordinate taxa resulting in an overall reduction in species richness by competitive exclusion (Bastida et al., 2021). Such evidence is also found in plants where dominant plant species suppress the diversity by preventing the establishment of other plants (Loreau and Hector, 2001; Rajaniemi, 2003; Paquette and Messier, 2011). Hence, such observation corroborates our findings, where low carbon content and reduced microbial biomass in L-site soil showed increased microbial diversity. Moreover, the elevated temperature due to global warming directly impacts soil microbial respiration and microbial activity, thereby altering soil carbon content (Frey et al., 2013; Xu et al., 2021). Precisely, the rise in temperature increases microbial activity and promotes the decomposition of soil organic matter, which depletes the labile carbon substrates (Karhu et al., 2014). In response to such environmental conditions, the microbial communities in soil either acclimate or alter in composition, increasing the microbial biomass (Allison and Martiny, 2008; Bradford, 2013). Hence, the observed difference in the microbial structure and activities between the two studied sites (L and P) can also be partially attributed to the temperature difference.
4.4. Relationship between the edaphic variables and the soil microbial communities
The soil organic matter represented by the TOC and TN content plays a vital role in shaping the soil microbiome (Drenovsky et al., 2004; Burns et al., 2015). Several studies reported that the amendment of organic carbon and nitrogen into the soil significantly influenced the microbial composition (Drenovsky et al., 2004; Ng et al., 2014; Zhou et al., 2017). Our data revealed that the contribution of the edaphic factors such as TOC and TN to the soil microbial communities was significantly higher compared to soil pH and conductivity (Figure 12). The relative abundance of the bacterial genera belonging to class- Rhizobiales, Acidobacteriales, Chthoniobacterales, Rhodospirillales, Ktedonobacterales, and Planctomycetales showed a significant positive correlation with the TOC and TN content. These bacterial orders mediate carbon and nitrogen cycling in soil (Barton et al., 2014; Jones, 2015; Köberl et al., 2020). Planctomycetales are facultative chemoorganotrophs specializing in carbohydrate metabolism (Fuerst, 1995), while Rhodospirillales and Rhizobiales fix nitrogen in the soil and maintain the carbon-to-nitrogen ratio (Hayat et al., 2010; Jones, 2015). The relative abundance of such bacterial species is also strongly correlated with the potential carbon mineralization rates (Jones, 2015). Chthoniobacterales contribute to carbon cycling by degrading complex carbohydrates, such as cellulose and xylan (Köberl et al., 2020), while Ktedonobacterales encode ureases and utilize nitrite and nitrate (Barton et al., 2014). Furthermore, the present study reveals a correlation between bacterial species and soil pH (Figure 10A). These bacterial species were also involved in nutrient cycling through the decomposition of organic matter and nitrogen fixation in soil (Wang et al., 2016).
Similarly, the relative abundance of fungal communities was positively correlated to the TOC, TN content and soil pH (Figure 10B). These fungal communities primarily contribute to carbohydrate degradation, thereby influencing the soil organic matter content and maintaining the nitrogen: phosphorus (N:P) ratio in soil (Kottke et al., 1998; Deacon et al., 2006; Avis, 2012; Ohm et al., 2012). Hence, our study revealed the impact of long-term precipitation variation complemented by soil texture and pH on the soil microfauna and their function in the Norway spruce forest. However, further studies at the metatranscriptomics and metaproteomics levels can validate our current findings at the functional level.
5. Conclusion
The present study evaluated the impact of long-term precipitation change over three decades on multiple edaphic variables and the microbial community structure in the soil. The combined effect of multiple edaphic factors strongly influences the soil microbial community composition and diversity. The bacterial and fungal communities in soil showed a significant relationship with soil pH, total organic carbon (TOC), and nitrogen (TN). Moreover, the soil metabolites, the extracellular enzyme activities, and PLFA content were primarily influenced by nutrient availability, indicating precipitation as a key driving factor. The variation in the soil microbial communities between the two seed orchards was also due to precipitation changes and a combination of several abiotic factors such as total organic carbon (TOC), total nitrogen (TN), and pH. Our study showed that increased precipitation complemented by soil texture enhances water and nutrient availability in soil, thereby increasing extracellular enzyme activities. Further, we observed that the microbial biomass and their activity are enhanced due to increased soil moisture content but showed low microbial diversity suggesting that soil microbial communities readily adapt to their environment.
Data availability statement
The datasets presented in this study can be found in online repositories. The names of the repository/repositories and accession number(s) can be found below: https://www.ncbi.nlm.nih.gov/, PRJNA766332 https://www.ncbi.nlm.nih.gov/, PRJNA766339.
Author contributions
AR conceptualize and supervise the study. DZ, JD, ZF, JK, and AR conducted fieldwork. AC and DZ conducted lab experiments. TC and PŽ conducted the PLFA assay and metabolomic study, respectively. AC and AR did the data analysis and wrote the first draft manuscript. All authors read and contributed to the final version of the manuscript.
Acknowledgments
This project was funded by the Internal Grant Agency (IGA) from the Faculty of Forestry and Wood Sciences, Czech University of Life sciences. Infrastructural support and salary for AC and AR are provided by grant “EVA 4.0,” No. CZ.02.1.01/0.0/0.0/16_019/0000803 financed and grant No. CZ.02.1.01/0.0/0.0/15_003/0000433, “EXTEMIT – K project,” financed by OP RDE. AR and AC are also supported by “Excellent Team Grants” (2023-2024) from FLD, CZU. The laboratory of Petr Baldrian (Institute of Microbiology, The Czech Academy of Sciences) was acknowledged for support during soil enzymatic activity study.
Conflict of interest
The authors declare that the research was conducted in the absence of any commercial or financial relationships that could be construed as a potential conflict of interest.
Publisher’s note
All claims expressed in this article are solely those of the authors and do not necessarily represent those of their affiliated organizations, or those of the publisher, the editors and the reviewers. Any product that may be evaluated in this article, or claim that may be made by its manufacturer, is not guaranteed or endorsed by the publisher.
Supplementary material
The Supplementary Material for this article can be found online at: https://www.frontiersin.org/articles/10.3389/ffgc.2023.1142979/full#supplementary-material
To preserve formating style the supplementary excels are denoted as table.xls.
Supplementary Figure 1 | Box plot representing (A) the average precipitation (B) the average temperature recorded over three decades (1991–2019) at two different sites (Lipova and Prenet).
Supplementary Figure 2 | Rarefaction Curves for (A) bacterial 16S amplicon sequencing (B) fungal ITS sequencing of soil samples from two different locations, Lipova (L) and Prenet (P). Different colors and symbols denote different samples.
Supplementary Figure 3 | Flower diagram representing (A) the presence of 1511 core bacterial OTUs shared among the different soil samples collected from Lipova (L) site (B) 944 bacterial OTUs present in Prenet (P) soil samples (C) the occurrence of 127 fungal OTUs that were common in the soil samples from L site and (D) 160 core fungal OTUs shared among the soils collected from P site.
Supplementary Figure 4 | The OTU tree representation of the bacterial abundance in (A) Lipova soil (B) Prenet soil using GraPhlAn. The predominance of Proteobacteria followed by Firmicutes, Actinobacteria and Acidobacteria was observed in both soil samples. Bacteroidetes were documented in Lipova soil, while Verrucomicrobia and Chloroflexi were observed in Prenet soil. The different taxonomic levels are indicated by the circle ranging from inside out, and the size of the circles denotes the species abundance. Different phyla are displayed in different colors. The solid circles denote the high abundance of the top 40 species.
Supplementary Figure 5 | The OTU tree representation of the fungal abundance in (A) Lipova soil (B) Prenet soil by using GraPhlAn. The predominance of Ascomycota, Basidiomycota and Zygomycota is observed in the soil samples. The circle from the inside out indicates the different taxonomic levels, and the size of the circles denotes the species abundance. Different phyla are displayed in different colors. The solid circles denote the high abundance of the top 40 species.
Supplementary Figure 6 | The evolutionary tree representing (A) the top 100 bacterial genera (B) the top 100 fungal genera present in soils from two different sites (Lipova and Prenet). Different colors of the branches indicate different phyla. The relative abundance of each genus in each soil is displayed outside the circle with different colors denoting different soil samples.
Supplementary Figure 7 | Heatmap representation of Weighted and Unweighted Unifrac distance matrices indicating the pairwise dissimilarity coefficient of (A) bacterial and (D) fungal communities between Lipova (L) and Prenet (P) soils where Weighted Unifrac distance is displayed above, and Unweighted Unifrac distance denoted below. Boxplot illustrates the beta diversity variation based on Weighted Unifrac distance matrices between soil (B) bacterial and (E) fungal communities Lipova and Prenet sites. The significance of the difference between the soil was analysed by Wilcox signed ranged test. Unweighted pair group method with arithmetic mean (UPGMA) tree cluster based on Weighted Unifrac distance indicating the similarity in (C) bacterial and (F) fungal communities in the soil samples collected from the same site in replicates. The relative abundance of soil bacterial and fungal communities at the phylum level is represented along with the UPGMA tree.
Supplementary Figure 8 | T-test analysis to determine the significant variation of (A) bacterial and (B) fungal communities at the phylum level in Lipova and Prenet soils. The last panel denotes the abundance of the phyla that significantly differs between the two soils. Each bar represents the mean value of the abundance at the phylum level in soil that is significantly different. The right panel denotes the confidential interval between the soils. The left-most part of each circle stands for the lower 95% confidential interval limit, while the right-most part is the upper limit. The centre of the circle stands for the difference in the mean value. The color of the circle resembles the soil sample, whose mean value is higher. The right-most value is the p-value of the significance test.
Supplementary Figure 9 | LEfSe analysis representing histogram of the LDA scores illustrating the presence of (A) bacterial and (B) fungal species (biomarker) whose abundance differs significantly between the soils from Lipova and Prenet. The length of each bin, i.e., LDA score, represents the effect size (the extent to which a biomarker can explain the differentiating phenotypes among groups) at the LDA score cutoff threshold >4.
Supplementary Table 1 | Analysis of Similarity (ANOSIM) representing the magnitude of variation in the bacterial and fungal communities between the two different forest soil samples. The positive R values indicate significant differences in the microbial communities in two different soils. P-value < 0.05 represents significant differences.
Supplementary Table 2 | MRPP Analysis representing the difference in the microbial communities (bacterial and fungal) in Lipova and Prenet soil samples. The less observed-delta value indicates low variation in bacterial and fungal communities within the biological replicates of each soil sample, while higher expected-delta values indicate larger differences between the soil samples. A positive A-value denotes that the difference between the two soil samples is larger than the difference within replicates. The significance value <0.05 indicates significant differences in the soil microbiota between the two samples.
Supplementary Table 3 | ADONIS Analysis based on the Bray-Curtis method indicates the significant difference between the bacterial and fungal communities between the two different forest soils. (Df - degree of freedom, MeanSqs- SS/Df, F. Model- F-test value, R2- the ratio of grouping variance and total variance). Values in parentheses denote Residual Error. The Pr value represents the significant variation in the microbial community structure.
Supplementary Table 4 | AMOVA Analysis determining differences in microbial community structure between the two forest soils. Df - denotes the degree of freedom, MeanSqs- SS/Df, F.Model- F-test value. Values in parentheses stand for Residual Error. The P-value determines the significant variation.
Supplementary Excel 1 | Soil metabolite profiling.
Supplementary Excel 2 | Bacterial_Raw_Clean_QC.
Supplementary Excel 3 | Fungal_Raw_Clean_QC.
Supplementary Excel 4 | Relative abundance of bacterial communities.
Supplementary Excel 5 | Relative abundance of fungal communities.
Supplementary Excel 6 | Core and unique soil bacterial communities_Lipova_Prenet.
Supplementary Excel 7 | Core and unique soil fungal communities_Lipova_Prenet.
Supplementary Excel 8 | Core and unique bacterial communities_Lipova soil.
Supplementary Excel 9 | Core and unique fungal communities_Lipova soil.
Supplementary Excel 10 | Core and unique bacterial communities_Prenet soil.
Supplementary Excel 11 | Core and unique fungal communities_Prenet soil.
Footnotes
- ^ www.metaboanalyst.ca
- ^ http://ccb.jhu.edu/software/FLASH/
- ^ http://qiime.org/index.html
- ^ http://www.drive5.com/usearch/manual/uchime_algo.html
- ^ http://www.arb-silva.de/
- ^ http://drive5.com/uparse/
- ^ http://www.drive5.com/muscle/
References
Aina, P., and Periaswamy, S. (1985). Estimating available water-holding capacity of western Nigerian soils from soil texture and bulk density, using core and sieved samples. Soil Sci. 140, 55–58. doi: 10.1097/00010694-198507000-00007
Algina, J., and Keselman, H. (1999). Comparing squared multiple correlation coefficients: examination of a confidence interval and a test significance. Psychol. Methods 4, 76–83. doi: 10.1037/1082-989X.4.1.76
Allison, S. D., and Martiny, J. B. (2008). Resistance, resilience, and redundancy in microbial communities. Proc. Natl. Acad. Sci. 105(Suppl._1), 11512–11519. doi: 10.1073/pnas.0801925105
Anderson, M. J. (2001). A new method for non-parametric multivariate analysis of variance. Austral Ecol. 26, 32–46. doi: 10.1046/j.1442-9993.2001.01070.x
Anthony, M. A., Crowther, T. W., Van Der Linde, S., Suz, L. M., Bidartondo, M. I., Cox, F., et al. (2022). Forest tree growth is linked to mycorrhizal fungal composition and function across Europe. ISME J. 16, 1327–1336. doi: 10.1038/s41396-021-01159-7
Austin, A. T., Yahdjian, L., Stark, J. M., Belnap, J., Porporato, A., Norton, U., et al. (2004). Water pulses and biogeochemical cycles in arid and semiarid ecosystems. Oecologia 141, 221–235. doi: 10.1007/s00442-004-1519-1
Avis, P. G. (2012). Ectomycorrhizal iconoclasts: the ITS rDNA diversity and nitrophilic tendencies of fetid Russula. Mycologia 104, 998–1007. doi: 10.3852/11-399
Baldrian, P. (2009). Microbial enzyme-catalyzed processes in soils and their analysis. Plant Soil Environ. 55, 370–378. doi: 10.17221/134/2009-PSE
Baldrian, P. (2017). Forest microbiome: diversity, complexity and dynamics. FEMS Microbiol. Rev. 41, 109–130. doi: 10.1093/femsre/fuw040
Baldrian, P., and Valášková, V. (2008). Degradation of cellulose by basidiomycetous fungi. FEMS Microbiol. Rev. 32, 501–521. doi: 10.1111/j.1574-6976.2008.00106.x
Banerjee, M. R., and Chapman, S. J. (1996). The significance of microbial biomass sulphur in soil. Biol. Fertility Soils 22, 116–125. doi: 10.1007/BF00384442
Barnard, R. L., Osborne, C. A., and Firestone, M. K. (2013). Responses of soil bacterial and fungal communities to extreme desiccation and rewetting. ISME J. 7, 2229–2241. doi: 10.1038/ismej.2013.104
Barton, H. A., Giarrizzo, J. G., Suarez, P., Robertson, C. E., Broering, M. J., Banks, E. D., et al. (2014). Microbial diversity in a Venezuelan orthoquartzite cave is dominated by the Chloroflexi (Class Ktedonobacterales) and Thaumarchaeota group I. 1c. Front. Microbiol. 5:615. doi: 10.3389/fmicb.2014.00615
Bastida, F., Eldridge, D. J., García, C., Png, G. K., Bardgett, R. D., and Delgado-Baquerizo, M. (2021). Soil microbial diversity–biomass relationships are driven by soil carbon content across global biomes. ISME J. 15, 2081–2091. doi: 10.1038/s41396-021-00906-0
Bastida, F., Torres, I. F., Andrés-Abellán, M., Baldrian, P., López-Mondéjar, R., Vìtrovskı, T., et al. (2017). Differential sensitivity of total and active soil microbial communities to drought and forest management. Glob. Change Biol. 23, 4185–4203. doi: 10.1111/gcb.13790
Bastida, F., Torres, I. F., Moreno, J. L., Baldrian, P., Ondoño, S., Ruiz-Navarro, A., et al. (2016). The active microbial diversity drives ecosystem multifunctionality and is physiologically related to carbon availability in Mediterranean semi-arid soils. Mol. Ecol. 25, 4660–4673. doi: 10.1111/mec.13783
Bechtold, J. S., and Naiman, R. J. (2006). Soil texture and nitrogen mineralization potential across a riparian toposequence in a semi-arid savanna. Soil Biol. Biochem. 38, 1325–1333. doi: 10.1016/j.soilbio.2005.09.028
Bell, C. W., Fricks, B. E., Rocca, J. D., Steinweg, J. M., McMahon, S. K., and Wallenstein, M. D. (2013). High-throughput fluorometric measurement of potential soil extracellular enzyme activities. J. Vis. Exp. 81:e50961. doi: 10.3791/50961
Bell, C. W., Tissue, D. T., Loik, M. E., Wallenstein, M. D., Acosta-Martinez, V., Erickson, R. A., et al. (2014). Soil microbial and nutrient responses to 7 years of seasonally altered precipitation in a Chihuahuan desert grassland. Glob. Change Biol. 20, 1657–1673. doi: 10.1111/gcb.12418
Berg, M., Kniese, J., and Verhoef, H. (1998). Dynamics and stratification of bacteria and fungi in the organic layers of a Scots pine forest soil. Biol. Fertility Soils 26, 313–322. doi: 10.1007/s003740050382
Berthrong, S. T., Buckley, D. H., and Drinkwater, L. E. (2013). Agricultural management and labile carbon additions affect soil microbial community structure and interact with carbon and nitrogen cycling. Microbial Ecol. 66, 158–170. doi: 10.1007/s00248-013-0225-0
Bokulich, N. A., Subramanian, S., Faith, J. J., Gevers, D., Gordon, J. I., Knight, R., et al. (2013). Quality-filtering vastly improves diversity estimates from Illumina amplicon sequencing. Nat. Methods 10, 57–59. doi: 10.1038/nmeth.2276
Borcard, D., Legendre, P., and Drapeau, P. (1992). Partialling out the spatial component of ecological variation. Ecology 73, 1045–1055. doi: 10.2307/1940179
Borken, W., and Matzner, E. (2009). Reappraisal of drying and wetting effects on C and N mineralization and fluxes in soils. Glob. Change Biol. 15, 808–824. doi: 10.1111/j.1365-2486.2008.01681.x
Borowik, A., and Wyszkowska, J. (2016). Soil moisture as a factor affecting the microbiological and biochemical activity of soil. Plant Soil Environ. 62, 250–255. doi: 10.17221/158/2016-PSE
Borowik, A., Wyszkowska, J., and Kucharski, J. (2022). Bacteria and soil enzymes supporting the valorization of forested soils. Materials 15:3287. doi: 10.3390/ma15093287
Bowd, E. J., Banks, S. C., Bissett, A., May, T. W., and Lindenmayer, D. B. (2022). Disturbance alters the forest soil microbiome. Mol. Ecol. 31, 419–447. doi: 10.1111/mec.16242
Bowden, R. D., Deem, L., Plante, A. F., Peltre, C., Nadelhoffer, K., and Lajtha, K. (2014). Litter input controls on soil carbon in a temperate deciduous forest. Soil Sci. Soc. Am. J. 78, S66–S75. doi: 10.2136/sssaj2013.09.0413nafsc
Bowles, T. M., Acosta-Martínez, V., Calderón, F., and Jackson, L. E. (2014). Soil enzyme activities, microbial communities, and carbon and nitrogen availability in organic agroecosystems across an intensively-managed agricultural landscape. Soil Biol. Biochem. 68, 252–262. doi: 10.1016/j.soilbio.2013.10.004
Bradford, M. A. (2013). Thermal adaptation of decomposer communities in warming soils. Front. Microbiol. 4:333. doi: 10.3389/fmicb.2013.00333
Bremner, J. M. (1996). Nitrogen-total. Methods Soil Anal. Part 3 Chem. Methods 5, 1085–1121. doi: 10.2136/sssabookser5.3.c37
Burke, I. C., Yonker, C. M., Parton, W. J., Cole, C. V., Flach, K., and Schimel, D. S. (1989). Texture, climate, and cultivation effects on soil organic matter content in US grassland soils. Soil Sci. Soc. Am. J. 53, 800–805. doi: 10.2136/sssaj1989.03615995005300030029x
Burns, K. N., Kluepfel, D. A., Strauss, S. L., Bokulich, N. A., Cantu, D., and Steenwerth, K. L. (2015). Vineyard soil bacterial diversity and composition revealed by 16S rRNA genes: differentiation by geographic features. Soil Biol. Biochem. 91, 232–247. doi: 10.1016/j.soilbio.2015.09.002
Burt, R. (2014). Soil Survey Staff. Soil Survey Field and Laboratory Methods Manual. Soil Survey Investigations Report 51. Washington, DC: US Department of Agriculture.
Cai, L. (2006). Multi-response permutation procedure as an alternative to the analysis of variance: an SPSS implementation. Behav. Res. Methods 38, 51–59. doi: 10.3758/BF03192749
Canarini, A., Carrillo, Y., Mariotte, P., Ingram, L., and Dijkstra, F. A. (2016). Soil microbial community resistance to drought and links to C stabilization in an Australian grassland. Soil Biol. Biochem. 103, 171–180. doi: 10.1016/j.soilbio.2016.08.024
Caporaso, J. G., Kuczynski, J., Stombaugh, J., Bittinger, K., Bushman, F. D., Costello, E. K., et al. (2010). QIIME allows analysis of high-throughput community sequencing data. Nat. Methods 7, 335–336. doi: 10.1038/nmeth.f.303
Cavigelli, M. A., Robertson, G. P., and Klug, M. J. (1995). “Fatty acid methyl ester (FAME) profiles as measures of soil microbial community structure,” in The significance and regulation of soil biodiversity. Developments in plant and soil sciences, Vol. 63, eds H. P. Collins, G. P. Robertson, and M. J. Klug (Dordrecht: Springer), 99–113. doi: 10.1007/978-94-011-0479-1_8
Chang, E.-H., Tian, G., and Chiu, C.-Y. (2017). Soil microbial communities in natural and managed cloud montane forests. Forests 8:33. doi: 10.3390/f8010033
Chao, A., Lee, S.-M., and Chen, T.-C. (1988). A generalized Good’s nonparametric coverage estimator. Chin. J. Math. 6, 189–199.
Chaparro, J. M., Sheflin, A. M., Manter, D. K., and Vivanco, J. M. (2012). Manipulating the soil microbiome to increase soil health and plant fertility. Biol. Fertility Soils 48, 489–499. doi: 10.1007/s00374-012-0691-4
Chen, X., Zhang, D., Liang, G., Qiu, Q., Liu, J., Zhou, G., et al. (2016). Effects of precipitation on soil organic carbon fractions in three subtropical forests in southern China. J. Plant Ecol. 9, 10–19. doi: 10.1093/jpe/rtv027
Chernov, T. I., Zhelezova, A. D., Tkhakakhova, A. K., Ksenofontova, N. A., Zverev, A. O., and Tiunov, A. V. (2021). Soil microbiome, organic matter content and microbial abundance in forest and forest-derived land cover in Cat Tien National Park (Vietnam). Appl. Soil Ecol. 165:103957. doi: 10.1016/j.apsoil.2021.103957
Chodak, M., Klimek, B., and Niklińska, M. (2016). Composition and activity of soil microbial communities in different types of temperate forests. Biol. Fertility Soils 52, 1093–1104. doi: 10.1007/s00374-016-1144-2
Chong, J., Wishart, D. S., and Xia, J. (2019). Using MetaboAnalyst 4.0 for comprehensive and integrative metabolomics data analysis. Curr. Protoc. Bioinformatics 68:e86. doi: 10.1002/cpbi.86
Clarke, K. R. (1993). Non-parametric multivariate analyses of changes in community structure. Aust. J. Ecol. 18, 117–143. doi: 10.1111/j.1442-9993.1993.tb00438.x
Collins, S. L., Belnap, J., Grimm, N., Rudgers, J., Dahm, C. N., D’odorico, P., et al. (2014). A multiscale, hierarchical model of pulse dynamics in arid-land ecosystems. Annu. Rev. Ecol. Evol. Syst. 45, 397–419. doi: 10.1146/annurev-ecolsys-120213-091650
Coolen, M. J., and Overmann, J. R. (2000). Functional exoenzymes as indicators of metabolically active bacteria in 124,000-year-old sapropel layers of the eastern Mediterranean Sea. Appl. Environ. Microbiol. 66, 2589–2598. doi: 10.1128/AEM.66.6.2589-2598.2000
Coûteaux, M.-M., Raubuch, M., and Berg, M. (1998). Response of protozoan and microbial communities in various coniferous forest soils after transfer to forests with different levels of atmospheric pollution. Biol. Fertility Soils 27, 179–188. doi: 10.1007/s003740050418
Crowther, T. W., Todd-Brown, K. E., Rowe, C. W., Wieder, W. R., Carey, J. C., Machmuller, M. B., et al. (2016). Quantifying global soil carbon losses in response to warming. Nature 540, 104–108. doi: 10.1038/nature20150
D’Argenio, V., Casaburi, G., Precone, V., and Salvatore, F. (2014). Comparative metagenomic analysis of human gut microbiome composition using two different bioinformatic pipelines. BioMed Res. Int. 2014:325340. doi: 10.1155/2014/325340
De Feudis, M., Cardelli, V., Massaccesi, L., Lagomarsino, A., Fornasier, F., Westphalen, D. J., et al. (2017). Influence of altitude on biochemical properties of European beech (Fagus sylvatica L.) forest soils. Forests 8:213. doi: 10.3390/f8060213
De Vries, F. T., Manning, P., Tallowin, J. R., Mortimer, S. R., Pilgrim, E. S., Harrison, K. A., et al. (2012). Abiotic drivers and plant traits explain landscape-scale patterns in soil microbial communities. Ecol. Lett. 15, 1230–1239. doi: 10.1111/j.1461-0248.2012.01844.x
De Vries, F. T., and Shade, A. (2013). Controls on soil microbial community stability under climate change. Front. Microbiol. 4:265. doi: 10.3389/fmicb.2013.00265
Deacon, L. J., Pryce-Miller, E. J., Frankland, J. C., Bainbridge, B. W., Moore, P. D., and Robinson, C. H. (2006). Diversity and function of decomposer fungi from a grassland soil. Soil Biol. Biochem. 38, 7–20. doi: 10.1016/j.soilbio.2005.04.013
Delgado-Baquerizo, M., Oliverio, A. M., Brewer, T. E., Benavent-González, A., Eldridge, D. J., Bardgett, R. D., et al. (2018). A global atlas of the dominant bacteria found in soil. Science 359, 320–325. doi: 10.1126/science.aap9516
Dexter, A. (2004). Soil physical quality: part I. theory, effects of soil texture, density, and organic matter, and effects on root growth. Geoderma 120, 201–214. doi: 10.1016/j.geoderma.2003.09.004
Drenovsky, R., Vo, D., Graham, K., and Scow, K. (2004). Soil water content and organic carbon availability are major determinants of soil microbial community composition. Microbial Ecol. 48, 424–430. doi: 10.1007/s00248-003-1063-2
Edgar, R. C. (2004). MUSCLE: multiple sequence alignment with high accuracy and high throughput. Nucleic Acids Res. 32, 1792–1797. doi: 10.1093/nar/gkh340
Edgar, R. C. (2013). UPARSE: highly accurate OTU sequences from microbial amplicon reads. Nat. Methods 10, 996–998. doi: 10.1038/nmeth.2604
Edgar, R. C., Haas, B. J., Clemente, J. C., Quince, C., and Knight, R. (2011). UCHIME improves sensitivity and speed of chimera detection. Bioinformatics 27, 2194–2200. doi: 10.1093/bioinformatics/btr381
el Zahar Haichar, F., Marol, C., Berge, O., Rangel-Castro, J. I., Prosser, J. I., Balesdent, J. M., et al. (2008). Plant host habitat and root exudates shape soil bacterial community structure. ISME J. 2, 1221–1230. doi: 10.1038/ismej.2008.80
Elbert, W., Weber, B., Burrows, S., Steinkamp, J., Büdel, B., Andreae, M. O., et al. (2012). Contribution of cryptogamic covers to the global cycles of carbon and nitrogen. Nat. Geosci. 5, 459–462. doi: 10.1038/ngeo1486
Fang, X., Zhou, G., Li, Y., Liu, S., Chu, G., Xu, Z., et al. (2016). Warming effects on biomass and composition of microbial communities and enzyme activities within soil aggregates in subtropical forest. Biol. Fertility Soils 52, 353–365. doi: 10.1007/s00374-015-1081-5
Fekete, I., Kotroczó, Z., Varga, C., Nagy, P. T., Várbíró, G., Bowden, R. D., et al. (2014). Alterations in forest detritus inputs influence soil carbon concentration and soil respiration in a central-European deciduous forest. Soil Biol. Biochem. 74, 106–114. doi: 10.1016/j.soilbio.2014.03.006
Fekete, I., Lajtha, K., Kotroczó, Z., Várbíró, G., Varga, C., Tóth, J. A., et al. (2017). Long-term effects of climate change on carbon storage and tree species composition in a dry deciduous forest. Glob. Change Biol. 23, 3154–3168. doi: 10.1111/gcb.13669
Fekete, I., Varga, C., Biró, B., Tóth, J. A., Várbíró, G., Lajtha, K., et al. (2016). The effects of litter production and litter depth on soil microclimate in a central european deciduous forest. Plant Soil 398, 291–300. doi: 10.1007/s11104-015-2664-5
Fierer, N. (2017). Embracing the unknown: disentangling the complexities of the soil microbiome. Nat. Rev. Microbiol. 15, 579–590. doi: 10.1038/nrmicro.2017.87
Fierer, N., and Jackson, R. B. (2006). The diversity and biogeography of soil bacterial communities. Proc. Natl. Acad. Sci. 103, 626–631. doi: 10.1073/pnas.0507535103
Fontaine, S., Barot, S., Barré, P., Bdioui, N., Mary, B., and Rumpel, C. (2007). Stability of organic carbon in deep soil layers controlled by fresh carbon supply. Nature 450, 277–280. doi: 10.1038/nature06275
Franzluebbers, A., Haney, R., Hons, F., and Zuberer, D. (1996). Active fractions of organic matter in soils with different texture. Soil Biol. Biochem. 28, 1367–1372. doi: 10.1016/S0038-0717(96)00143-5
Frey, B., Carnol, M., Dharmarajah, A., Brunner, I., and Schleppi, P. (2020). Only minor changes in the soil microbiome of a Sub-alpine forest after 20 years of moderately increased nitrogen loads. Front. For. Glob. Change 3:77. doi: 10.3389/ffgc.2020.00077
Frey, S. D., Lee, J., Melillo, J. M., and Six, J. (2013). The temperature response of soil microbial efficiency and its feedback to climate. Nat. Clim. Change 3, 395–398. doi: 10.1038/nclimate1796
Fuerst, J. A. (1995). The planctomycetes: emerging models for microbial ecology, evolution and cell biology. Microbiology 141, 1493–1506. doi: 10.1099/13500872-141-7-1493
Gao, W., Kou, L., Zhang, J., Müller, C., Wang, H., Yang, H., et al. (2016). Enhanced deposition of nitrate alters microbial cycling of N in a subtropical forest soil. Biol. Fertility Soils 52, 977–986. doi: 10.1007/s00374-016-1134-4
Haas, B. J., Gevers, D., Earl, A. M., Feldgarden, M., Ward, D. V., Giannoukos, G., et al. (2011). Chimeric 16S rRNA sequence formation and detection in Sanger and 454-pyrosequenced PCR amplicons. Genome Res. 21, 494–504. doi: 10.1101/gr.112730.110
Haas, J. C., Street, N. R., Sjödin, A., Lee, N. M., Högberg, M. N., Näsholm, T., et al. (2018). Microbial community response to growing season and plant nutrient optimisation in a boreal Norway spruce forest. Soil Biol. Biochem. 125, 197–209. doi: 10.1016/j.soilbio.2018.07.005
Harris, R. (1981). Effect of water potential on microbial growth and activity. Water Potential Relations Soil Microbiol. 9, 23–95. doi: 10.2136/sssaspecpub9.c2
Hayat, R., Ali, S., Amara, U., Khalid, R., and Ahmed, I. (2010). Soil beneficial bacteria and their role in plant growth promotion: a review. Ann. Microbiol. 60, 579–598. doi: 10.1007/s13213-010-0117-1
He, R., Yang, K., Li, Z., Schädler, M., Yang, W., Wu, F., et al. (2017). Effects of forest conversion on soil microbial communities depend on soil layer on the eastern Tibetan Plateau of China. PLoS One 12:e0186053. doi: 10.1371/journal.pone.0186053
Horz, H.-P., Barbrook, A., Field, C. B., and Bohannan, B. J. (2004). Ammonia-oxidizing bacteria respond to multifactorial global change. Proc. Natl. Acad. Sci. 101, 15136–15141. doi: 10.1073/pnas.0406616101
Huang, G., Li, Y., and Su, Y. G. (2015). Effects of increasing precipitation on soil microbial community composition and soil respiration in a temperate desert, Northwestern China. Soil Biol. Biochem. 83, 52–56. doi: 10.1016/j.soilbio.2015.01.007
Hueso, S., García, C., and Hernández, T. (2012). Severe drought conditions modify the microbial community structure, size and activity in amended and unamended soils. Soil Biol. Biochem. 50, 167–173. doi: 10.1016/j.soilbio.2012.03.026
Huhta, V., and Setälä, H. (1990). Laboratory design to simulate complexity of forest floor for studying the role of fauna in the soil processes. Biol. Fertility Soils 10, 155–162. doi: 10.1007/BF00336129
Hultman, J., Waldrop, M. P., Mackelprang, R., David, M. M., McFarland, J., Blazewicz, S. J., et al. (2015). Multi-omics of permafrost, active layer and thermokarst bog soil microbiomes. Nature 521, 208–212. doi: 10.1038/nature14238
Hungate, B. A., Hart, S. C., Selmants, P. C., Boyle, S. I., and Gehring, C. A. (2007). Soil responses to management, increased precipitation, and added nitrogen in ponderosa pine forests. Ecol. Appl. 17, 1352–1365. doi: 10.1890/06-1187.1
Johns, C. W., Lee, A. B., Springer, T. I., Rosskopf, E. N., Hong, J. C., Turechek, W., et al. (2017). Using NMR-based metabolomics to monitor the biochemical composition of agricultural soils: a pilot study. Eur. J. Soil Biol. 83, 98–105. doi: 10.1016/j.ejsobi.2017.10.008
Jones, O. A., Sdepanian, S., Lofts, S., Svendsen, C., Spurgeon, D. J., Maguire, M. L., et al. (2014). Metabolomic analysis of soil communities can be used for pollution assessment. Environ. Toxicol. Chem. 33, 61–64. doi: 10.1002/etc.2418
Jones, R.T. (2015). “A comprehensive survey of soil rhizobiales diversity using high-throughput DNA sequencing,” in Biological nitrogen fixation, Vol 2, ed. F.J. Bruijn (New Jersey, NJ: John Wiley & Sons, Inc.), 769–776.
Judd, K. E., Crump, B. C., and Kling, G. W. (2006). Variation in dissolved organic matter controls bacterial production and community composition. Ecology 87, 2068–2079. doi: 10.1890/0012-9658(2006)87[2068:VIDOMC]2.0.CO;2
Kanehisa, M., Goto, S., Sato, Y., Furumichi, M., and Tanabe, M. (2012). KEGG for integration and interpretation of large-scale molecular data sets. Nucleic Acids Res. 40, D109–D114. doi: 10.1093/nar/gkr988
Karhu, K., Auffret, M. D., Dungait, J. A., Hopkins, D. W., Prosser, J. I., Singh, B. K., et al. (2014). Temperature sensitivity of soil respiration rates enhanced by microbial community response. Nature 513, 81–84. doi: 10.1038/nature13604
Kennedy, P. G., and Maillard, F. (2022). Knowns and unknowns of the soil fungal necrobiome. Trends in Microbiol. 31, 173–180. doi: 10.1016/j.tim.2022.08.011
Kieft, T. L. (1987). Microbial biomass response to a rapid increase in water potential when dry soil is wetted. Soil Biol. Biochem. 19, 119–126. doi: 10.1016/0038-0717(87)90070-8
Klamer, M., and Bååth, E. (1998). Microbial community dynamics during composting of straw material studied using phospholipid fatty acid analysis. FEMS Microbiol. Ecol. 27, 9–20. doi: 10.1111/j.1574-6941.1998.tb00521.x
Klindworth, A., Pruesse, E., Schweer, T., Peplies, J., Quast, C., Horn, M., et al. (2013). Evaluation of general 16S ribosomal RNA gene PCR primers for classical and next-generation sequencing-based diversity studies. Nucleic Acids Res. 41:e1. doi: 10.1093/nar/gks808
Knapp, A. K., Ciais, P., and Smith, M. D. (2017). Reconciling inconsistencies in precipitation–productivity relationships: implications for climate change. New Phytol. 214, 41–47. doi: 10.1111/nph.14381
Köberl, M., Wagner, P., Müller, H., Matzer, R., Unterfrauner, H., Cernava, T., et al. (2020). Unraveling the complexity of soil microbiomes in a large-scale study subjected to different agricultural management in Styria. Front. Microbiol. 11:1052. doi: 10.3389/fmicb.2020.01052
Kottke, I., Qian, X. M., Pritsch, K., Haug, I., and Oberwinkler, F. (1998). Xerocomus badius–Picea abies, an ectomycorrhiza of high activity and element storage capacity in acidic soil. Mycorrhiza 7, 267–275. doi: 10.1007/s005720050191
Krashevska, V., Klarner, B., Widyastuti, R., Maraun, M., and Scheu, S. (2015). Impact of tropical lowland rainforest conversion into rubber and oil palm plantations on soil microbial communities. Biol. Fertility Soils 51, 697–705. doi: 10.1007/s00374-015-1021-4
Kuzyakov, Y. (2010). Priming effects: interactions between living and dead organic matter. Soil Biol. Biochem. 42, 1363–1371. doi: 10.1016/j.soilbio.2010.04.003
Lankadurai, B. P., Nagato, E. G., and Simpson, M. J. (2013). Environmental metabolomics: an emerging approach to study organism responses to environmental stressors. Environ. Rev. 21, 180–205. doi: 10.1139/er-2013-0011
Lê Cao, K.-A., Boitard, S., and Besse, P. (2011). Sparse PLS discriminant analysis: biologically relevant feature selection and graphical displays for multiclass problems. BMC Bioinformatics 12:253. doi: 10.1186/1471-2105-12-253
Legendre, P. (2008). Studying beta diversity: ecological variation partitioning by multiple regression and canonical analysis. J. Plant Ecol. 1, 3–8. doi: 10.1093/jpe/rtm001
Li, H., Xu, Z., Yang, S., Li, X., Top, E. M., Wang, R., et al. (2016). Responses of soil bacterial communities to nitrogen deposition and precipitation increment are closely linked with aboveground community variation. Microbial Ecol. 71, 974–989. doi: 10.1007/s00248-016-0730-z
Li, H., Yang, S., Xu, Z., Yan, Q., Li, X., van Nostrand, J. D., et al. (2017). Responses of soil microbial functional genes to global changes are indirectly influenced by aboveground plant biomass variation. Soil Biol. Biochem. 104, 18–29. doi: 10.1016/j.soilbio.2016.10.009
Li, H., Ye, D., Wang, X., Settles, M. L., Wang, J., Hao, Z., et al. (2014). Soil bacterial communities of different natural forest types in Northeast China. Plant Soil 383, 203–216. doi: 10.1007/s11104-014-2165-y
Li, J., Li, Z., Wang, F., Zou, B., Chen, Y., Zhao, J., et al. (2015). Effects of nitrogen and phosphorus addition on soil microbial community in a secondary tropical forest of China. Biol. Fertility Soils 51, 207–215. doi: 10.1007/s00374-014-0964-1
Li, X., Qu, Z., Zhang, Y., Ge, Y., and Sun, H. (2022). Soil fungal community and potential function in different forest ecosystems. Diversity 14:520. doi: 10.3390/d14070520
Li, Z., Wu, P., Feng, H., Zhao, X., and Huang, J. (2009). Effects of soil clay particle content on soil infiltration capacity by simulated experiments. Agric. Res. Arid Areas 27, 71–77.
Linn, D. M., and Doran, J. W. (1984). Effect of water-filled pore space on carbon dioxide and nitrous oxide production in tilled and nontilled soils. Soil Sci. Soc. Am. J. 48, 1267–1272. doi: 10.2136/sssaj1984.03615995004800060013x
Lisá, L., Bajer, A., Pacina, J., McCool, J.-P., Cílek, V., Rohovec, J., et al. (2017). Prehistoric dark soils/sediments of Central Sudan; case study from the Mesolithic landscape at the Sixth Nile Cataract. Catena 149, 273–282. doi: 10.1016/j.catena.2016.09.023
Liu, W., Zhang, Z., and Wan, S. (2009). Predominant role of water in regulating soil and microbial respiration and their responses to climate change in a semiarid grassland. Glob. Change Biol. 15, 184–195. doi: 10.1111/j.1365-2486.2008.01728.x
Liu, Y., Liu, S., Wan, S., Wang, J., Luan, J., and Wang, H. (2016). Differential responses of soil respiration to soil warming and experimental throughfall reduction in a transitional oak forest in central China. Agric. For. Meteorol. 226, 186–198. doi: 10.1016/j.agrformet.2016.06.003
Lladó, S., López-Mondéjar, R., and Baldrian, P. (2017). Forest soil bacteria: diversity, involvement in ecosystem processes, and response to global change. Microbiol. Mol. Biol. Rev. 81:e00063-16. doi: 10.1128/MMBR.00063-16
Loreau, M., and Hector, A. (2001). Partitioning selection and complementarity in biodiversity experiments. Nature 412, 72–76. doi: 10.1038/35083573
Lozupone, C., Lladser, M. E., Knights, D., Stombaugh, J., and Knight, R. (2011). UniFrac: an effective distance metric for microbial community comparison. ISME J. 5, 169–172. doi: 10.1038/ismej.2010.133
Lozupone, C. A., Hamady, M., Kelley, S. T., and Knight, R. (2007). Quantitative and qualitative β diversity measures lead to different insights into factors that structure microbial communities. Appl. Environ. Microbiol. 73, 1576–1585. doi: 10.1128/AEM.01996-06
Maestre, F. T., Delgado-Baquerizo, M., Jeffries, T. C., Eldridge, D. J., Ochoa, V., Gozalo, B., et al. (2015). Increasing aridity reduces soil microbial diversity and abundance in global drylands. Proc. Natl. Acad. Sci. 112, 15684–15689. doi: 10.1073/pnas.1516684112
Maestre, F. T., Eldridge, D. J., Soliveres, S., Kéfi, S., Delgado-Baquerizo, M., Bowker, M. A., et al. (2016). Structure and functioning of dryland ecosystems in a changing world. Annu. Rev. Ecol. Evol. Syst. 47, 215–237. doi: 10.1146/annurev-ecolsys-121415-032311
Magoè, T., and Salzberg, S. L. (2011). FLASH: fast length adjustment of short reads to improve genome assemblies. Bioinformatics 27, 2957–2963. doi: 10.1093/bioinformatics/btr507
Magurran, A. E. (1988). Ecological Diversity and Its Measurement. Princeton, NJ: Princeton university press. doi: 10.1007/978-94-015-7358-0
Manzoni, S., Taylor, P., Richter, A., Porporato, A., and Ågren, G. I. (2012). Environmental and stoichiometric controls on microbial carbon-use efficiency in soils. New Phytol. 196, 79–91. doi: 10.1111/j.1469-8137.2012.04225.x
Martin, P. H., Sherman, R. E., and Fahey, T. J. (2004). Forty years of tropical forest recovery from agriculture: structure and floristics of secondary and old-growth riparian forests in the Dominican Republic. Biotropica 36, 297–317. doi: 10.1111/j.1744-7429.2004.tb00322.x
Martinoviæ, T., Mašínová, T., López-Mondéjar, R., Jansa, J., Štursová, M., Starke, R., et al. (2022). Microbial utilization of simple and complex carbon compounds in a temperate forest soil. Soil Biol. Biochem. 173:108786. doi: 10.1016/j.soilbio.2022.108786
Maxwell, T. L., Augusto, L., Bon, L., Courbineau, A., Altinalmazis-Kondylis, A., Milin, S., et al. (2020). Effect of a tree mixture and water availability on soil nutrients and extracellular enzyme activities along the soil profile in an experimental forest. Soil Biol. Biochem. 148:107864. doi: 10.1016/j.soilbio.2020.107864
Meena, M., Yadav, G., Sonigra, P., Nagda, A., Mehta, T., Swapnil, P., et al. (2022). Multifarious responses of forest soil microbial community toward climate change. Microb. Ecol. Online ahead of print. doi: 10.1007/s00248-022-02051-3
Meier, I. C., and Leuschner, C. (2010). Variation of soil and biomass carbon pools in beech forests across a precipitation gradient. Glob. Change Biol. 16, 1035–1045. doi: 10.1111/j.1365-2486.2009.02074.x
Micallef, S. A., Shiaris, M. P., and Colón-Carmona, A. (2009). Influence of Arabidopsis thaliana accessions on rhizobacterial communities and natural variation in root exudates. J. Exp. Bot. 60, 1729–1742. doi: 10.1093/jxb/erp053
Mishra, A., Singh, L., and Singh, D. (2022). Unboxing the black box—one step forward to understand the soil microbiome: a systematic review. Microb. Ecol. 85, 669–683. doi: 10.1007/s00248-022-01962-5
Moore-Kucera, J., and Dick, R. P. (2008). PLFA profiling of microbial community structure and seasonal shifts in soils of a Douglas-fir chronosequence. Microb. Ecol. 55, 500–511. doi: 10.1007/s00248-007-9295-1
Naylor, D., Sadler, N., Bhattacharjee, A., Graham, E. B., Anderton, C. R., McClure, R., et al. (2020). Soil microbiomes under climate change and implications for carbon cycling. Annu. Rev. Environ. Resour. 45, 29–59. doi: 10.1146/annurev-environ-012320-082720
Nelson, D., and Sommers, L. E. (1983). “Total carbon, organic carbon, and organic matter,” in Methods of Soil Analysis: Part 2 Chemical and Microbiological Properties, ed. A. L. Page (Hoboken NJ: Wiley).
Ng, E.-L., Patti, A., Rose, M., Schefe, C., Wilkinson, K., Smernik, R., et al. (2014). Does the chemical nature of soil carbon drive the structure and functioning of soil microbial communities? Soil Biol. Biochem. 70, 54–61. doi: 10.1016/j.soilbio.2013.12.004
Nielsen, U. N., and Ball, B. A. (2015). Impacts of altered precipitation regimes on soil communities and biogeochemistry in arid and semi-arid ecosystems. Glob. Change Biol. 21, 1407–1421. doi: 10.1111/gcb.12789
Nilsson, R. H., Larsson, K.-H., Taylor, A. F. S., Bengtsson-Palme, J., Jeppesen, T. S., Schigel, D., et al. (2019). The UNITE database for molecular identification of fungi: handling dark taxa and parallel taxonomic classifications. Nucleic Acids Res. 47, D259–D264. doi: 10.1093/nar/gky1022
O’brien, S. L., Jastrow, J. D., Grimley, D. A., and Gonzalez-Meler, M. A. (2010). Moisture and vegetation controls on decadal-scale accrual of soil organic carbon and total nitrogen in restored grasslands. Glob. Change Biol. 16, 2573–2588. doi: 10.1111/j.1365-2486.2009.02114.x
Ohm, R. A., Feau, N., Henrissat, B., Schoch, C. L., Horwitz, B. A., Barry, K. W., et al. (2012). Diverse lifestyles and strategies of plant pathogenesis encoded in the genomes of eighteen Dothideomycetes fungi. PLoS Pathogens 8:e1003037. doi: 10.1371/journal.ppat.1003037
Oksanen, J., Blanchet, F., Kindt, R., Legendre, P., O’hara, R., Simpson, G., et al. (2010). Vegan: Community Ecology Package. R Package Version 1.17-4.
Ostertag, R., Marín-Spiotta, E., Silver, W. L., and Schulten, J. (2008). Litterfall and decomposition in relation to soil carbon pools along a secondary forest chronosequence in Puerto Rico. Ecosystems 11, 701–714. doi: 10.1007/s10021-008-9152-1
Overy, D. P., Bell, M. A., Habtewold, J., Helgason, B. L., and Gregorich, E. G. (2021). Omics” technologies for the study of soil carbon stabilization: a review. Front. Environ. Sci. 9:617952. doi: 10.3389/fenvs.2021.617952
Paquette, A., and Messier, C. (2011). The effect of biodiversity on tree productivity: from temperate to boreal forests. Glob. Ecol. Biogeogr. 20, 170–180. doi: 10.1111/j.1466-8238.2010.00592.x
Patel, K. F., Fansler, S. J., Campbell, T. P., Bond-Lamberty, B., Smith, A. P., RoyChowdhury, T., et al. (2021). Soil texture and environmental conditions influence the biogeochemical responses of soils to drought and flooding. Commun. Earth Environ. 2:127. doi: 10.1038/s43247-021-00198-4
Paulson, J. N., Pop, M., and Bravo, H. C. (2011). Metastats: an improved statistical method for analysis of metagenomic data. Genome Biol. 12, 1–27. doi: 10.1186/1465-6906-12-S1-P17
Peres-Neto, P. R., Legendre, P., Dray, S., and Borcard, D. (2006). Variation partitioning of species data matrices: estimation and comparison of fractions. Ecology 87, 2614–2625. doi: 10.1890/0012-9658(2006)87[2614:VPOSDM]2.0.CO;2
Pétriacq, P., Williams, A., Cotton, A., McFarlane, A. E., Rolfe, S. A., and Ton, J. (2017). Metabolite profiling of non-sterile rhizosphere soil. Plant J. 92, 147–162. doi: 10.1111/tpj.13639
R Core Team (2013). R: a Language and Environment for Statistical Computing. Vienna: R Statistical Computing.
Raiesi, F. (2006). Carbon and N mineralization as affected by soil cultivation and crop residue in a calcareous wetland ecosystem in Central Iran. Agric. Ecosyst. Environ. 112, 13–20. doi: 10.1016/j.agee.2005.07.002
Rajaniemi, T. K. (2003). Explaining productivity-diversity relationships in plants. Oikos 101, 449–457. doi: 10.1034/j.1600-0706.2003.12128.x
Romaní, A. M., Fischer, H., Mille-Lindblom, C., and Tranvik, L. J. (2006). Interactions of bacteria and fungi on decomposing litter: differential extracellular enzyme activities. Ecology 87, 2559–2569. doi: 10.1890/0012-9658(2006)87[2559:IOBAFO]2.0.CO;2
Rousk, J., Bååth, E., Brookes, P. C., Lauber, C. L., Lozupone, C., Caporaso, J. G., et al. (2010). Soil bacterial and fungal communities across a pH gradient in an arable soil. ISME J. 4, 1340–1351. doi: 10.1038/ismej.2010.58
Rousk, J., Smith, A. R., and Jones, D. L. (2013). Investigating the long-term legacy of drought and warming on the soil microbial community across five E uropean shrubland ecosystems. Glob. Change Biol. 19, 3872–3884. doi: 10.1111/gcb.12338
Sayer, E. J., Wagner, M., Oliver, A. E., Pywell, R. F., James, P., Whiteley, A. S., et al. (2013). Grassland management influences spatial patterns of soil microbial communities. Soil Biol. Biochem. 61, 61–68. doi: 10.1016/j.soilbio.2013.02.012
Schimel, J. (2016). Microbial ecology: linking omics to biogeochemistry. Nat. Microbiol. 1:15028. doi: 10.1038/nmicrobiol.2015.28
Schimel, J., Balser, T. C., and Wallenstein, M. (2007). Microbial stress-response physiology and its implications for ecosystem function. Ecology 88, 1386–1394. doi: 10.1890/06-0219
Schimel, J. P. (2018). Life in dry soils: effects of drought on soil microbial communities and processes. Annu. Rev. Ecol. Evol. Syst. 49, 409–432. doi: 10.1146/annurev-ecolsys-110617-062614
Segata, N., Izard, J., Waldron, L., Gevers, D., Miropolsky, L., Garrett, W. S., et al. (2011). Metagenomic biomarker discovery and explanation. Genome Biol. 12:R60. doi: 10.1186/gb-2011-12-6-r60
Sheik, C. S., Mitchell, T. W., Rizvi, F. Z., Rehman, Y., Faisal, M., Hasnain, S., et al. (2012). Exposure of soil microbial communities to chromium and arsenic alters their diversity and structure. PLoS One 7:e40059. doi: 10.1371/journal.pone.0040059
Silver, W. L., Neff, J., McGroddy, M., Veldkamp, E., Keller, M., and Cosme, R. (2000). Effects of soil texture on belowground carbon and nutrient storage in a lowland Amazonian forest ecosystem. Ecosystems 3, 193–209. doi: 10.1007/s100210000019
Sinsabaugh, R. L. (2010). Phenol oxidase, peroxidase and organic matter dynamics of soil. Soil Biol. Biochem. 42, 391–404. doi: 10.1016/j.soilbio.2009.10.014
Sinsabaugh, R. L., Antibus, R. K., and Linkins, A. E. (1991). An enzymic approach to the analysis of microbial activity during plant litter decomposition. Agric. Ecosyst. Environ. 34, 43–54. doi: 10.1016/0167-8809(91)90092-C
Sinsabaugh, R. L., Lauber, C. L., Weintraub, M. N., Ahmed, B., Allison, S. D., Crenshaw, C., et al. (2008). Stoichiometry of soil enzyme activity at global scale. Ecol. Lett. 11, 1252–1264. doi: 10.1111/j.1461-0248.2008.01245.x
Six, J., Conant, R. T., Paul, E. A., and Paustian, K. (2002). Stabilization mechanisms of soil organic matter: implications for C-saturation of soils. Plant Soil 241, 155–176. doi: 10.1023/A:1016125726789
Six, J., Elliott, E. T., and Paustian, K. (2000). Soil macroaggregate turnover and microaggregate formation: a mechanism for C sequestration under no-tillage agriculture. Soil Biol. Biochem. 32, 2099–2103. doi: 10.1016/S0038-0717(00)00179-6
Slessarev, E., Lin, Y., Bingham, N., Johnson, J., Dai, Y., Schimel, J., et al. (2016). Water balance creates a threshold in soil pH at the global scale. Nature 540, 567–569. doi: 10.1038/nature20139
Šnajdr, J., Valášková, V., Merhautová, V. R., Herinková, J., Cajthaml, T., and Baldrian, P. (2008). Spatial variability of enzyme activities and microbial biomass in the upper layers of Quercus petraea forest soil. Soil Biol. Biochem. 40, 2068–2075. doi: 10.1016/j.soilbio.2008.01.015
Song, Y., Li, X., Yao, S., Yang, X., and Jiang, X. (2020). Correlations between soil metabolomics and bacterial community structures in the pepper rhizosphere under plastic greenhouse cultivation. Sci. Total Environ. 728:138439. doi: 10.1016/j.scitotenv.2020.138439
Sorensen, P. O., Germino, M. J., and Feris, K. P. (2013). Microbial community responses to 17 years of altered precipitation are seasonally dependent and coupled to co-varying effects of water content on vegetation and soil C. Soil Biol. Biochem. 64, 155–163. doi: 10.1016/j.soilbio.2013.04.014
Sridhar, B., Lawrence, G. B., Debenport, S. J., Fahey, T. J., Buckley, D. H., Wilhelm, R. C., et al. (2022). Watershed-scale liming reveals the short-and long-term effects of pH on the forest soil microbiome and carbon cycling. Environ. Microbiol. 24, 6184–6199. doi: 10.1111/1462-2920.16119
Stella, T., Covino, S., Burianová, E., Filipová, A., Křesinová, Z., Voříšková, J., et al. (2015). Chemical and microbiological characterization of an aged PCB-contaminated soil. Sci. Total Environ. 533, 177–186. doi: 10.1016/j.scitotenv.2015.06.019
Stocker, T. (2014). Climate Change 2013: the Physical Science Basis: Working Group I Contribution to the Fifth Assessment Report of the Intergovernmental Panel on Climate Change. Cambridge: Cambridge university press.
Su, X., Su, X., Yang, S., Zhou, G., Ni, M., Wang, C., et al. (2020). Drought changed soil organic carbon composition and bacterial carbon metabolizing patterns in a subtropical evergreen forest. Sci. Total Environ. 736:139568. doi: 10.1016/j.scitotenv.2020.139568
Swenson, T. L., Jenkins, S., Bowen, B. P., and Northen, T. R. (2015). Untargeted soil metabolomics methods for analysis of extractable organic matter. Soil Biol. Biochem. 80, 189–198. doi: 10.1016/j.soilbio.2014.10.007
Swenson, T. L., and Northen, T. R. (2019). Untargeted soil metabolomics using liquid chromatography–mass spectrometry and gas chromatography–mass spectrometry. Methods Mol. Biol. 1859, 97–109. doi: 10.1007/978-1-4939-8757-3_4
Tedersoo, L., Bahram, M., Põlme, S., Kõljalg, U., Yorou, N. S., Wijesundera, R., et al. (2014). Global diversity and geography of soil fungi. Science 346:1256688.
Tiemann, L. K., and Billings, S. A. (2011). Changes in variability of soil moisture alter microbial community C and N resource use. Soil Biol. Biochem. 43, 1837–1847. doi: 10.1016/j.soilbio.2011.04.020
Torsvik, V., and Øvreås, L. (2002). Microbial diversity and function in soil: from genes to ecosystems. Curr. Opin. Microbiol. 5, 240–245. doi: 10.1016/S1369-5274(02)00324-7
Treseder, K. K., Marusenko, Y., Romero-Olivares, A. L., and Maltz, M. R. (2016). Experimental warming alters potential function of the fungal community in boreal forest. Glob. Change Biol. 22, 3395–3404. doi: 10.1111/gcb.13238
Uroz, S., Ioannidis, P., Lengelle, J., Cébron, A., Morin, E., Buée, M., et al. (2013). Functional assays and metagenomic analyses reveals differences between the microbial communities inhabiting the soil horizons of a Norway spruce plantation. PLoS One 8:e55929. doi: 10.1371/journal.pone.0055929
Veneklaas, E. J., Stevens, J., Cawthray, G. R., Turner, S., Grigg, A. M., and Lambers, H. (2003). Chickpea and white lupin rhizosphere carboxylates vary with soil properties and enhance phosphorus uptake. Plant Soil 248, 187–197. doi: 10.1023/A:1022367312851
Vepsäläinen, M., Kukkonen, S., Vestberg, M., Sirviö, H., and Niemi, R. M. (2001). Application of soil enzyme activity test kit in a field experiment. Soil Biol. Biochem. 33, 1665–1672. doi: 10.1016/S0038-0717(01)00087-6
Vereecken, H., Maes, J., Feyen, J., and Darius, P. (1989). Estimating the soil moisture retention characteristic from texture, bulk density, and carbon content. Soil Sci. 148, 389–403. doi: 10.1097/00010694-198912000-00001
Wagai, R., Kitayama, K., Satomura, T., Fujinuma, R., and Balser, T. (2011). Interactive influences of climate and parent material on soil microbial community structure in Bornean tropical forest ecosystems. Ecol. Res. 26, 627–636. doi: 10.1007/s11284-011-0822-7
Wang, B., Chen, Y., Li, Y., Zhang, H., Yue, K., Wang, X., et al. (2021). Differential effects of altered precipitation regimes on soil carbon cycles in arid versus humid terrestrial ecosystems. Glob. Change Biol. 27, 6348–6362. doi: 10.1111/gcb.15875
Wang, M., Shi, S., Lin, F., and Jiang, P. (2014). Response of the soil fungal community to multi-factor environmental changes in a temperate forest. Appl. Soil Ecol. 81, 45–56. doi: 10.1016/j.apsoil.2014.04.008
Wang, Q., Garrity, G. M., Tiedje, J. M., and Cole, J. R. (2007). Naive Bayesian classifier for rapid assignment of rRNA sequences into the new bacterial taxonomy. Appl. Environ. Microbiol. 73, 5261–5267. doi: 10.1128/AEM.00062-07
Wang, W., Wang, H., Feng, Y., Wang, L., Xiao, X., Xi, Y., et al. (2016). Consistent responses of the microbial community structure to organic farming along the middle and lower reaches of the Yangtze River. Sci. Rep. 6:35046. doi: 10.1038/srep35046
Wang, X.-Q., Zhang, X.-C., Pei, X.-J., and Ren, G.-F. (2022). Effect of the particle size composition and dry density on the water retention characteristics of remolded loess. Minerals 12:698. doi: 10.3390/min12060698
Wentworth, C. K. (1922). A scale of grade and class terms for clastic sediments. J. Geol. 30, 377–392. doi: 10.1086/622910
White, I. R. A., Rivas-Ubach, A., Borkum, M. I., Köberl, M., Bilbao, A., Colby, S. M., et al. (2017). The state of rhizospheric science in the era of multi-omics: a practical guide to omics technologies. Rhizosphere 3, 212–221. doi: 10.1016/j.rhisph.2017.05.003
White, J. R., Nagarajan, N., and Pop, M. (2009). Statistical methods for detecting differentially abundant features in clinical metagenomic samples. PLoS Comput. Biol. 5:e1000352. doi: 10.1371/journal.pcbi.1000352
White, T. J., Bruns, T., Lee, S., and Taylor, J. (1990). Amplification and direct sequencing of fungal ribosomal RNA genes for phylogenetics. PCR Protoc. Guide Methods Appl. 18, 315–322. doi: 10.1016/B978-0-12-372180-8.50042-1
Wilcox, K. R., Shi, Z., Gherardi, L. A., Lemoine, N. P., Koerner, S. E., Hoover, D. L., et al. (2017). Asymmetric responses of primary productivity to precipitation extremes: a synthesis of grassland precipitation manipulation experiments. Glob. Change Biol. 23, 4376–4385. doi: 10.1111/gcb.13706
Williams, M. A., and Rice, C. W. (2007). Seven years of enhanced water availability influences the physiological, structural, and functional attributes of a soil microbial community. Appl. Soil Ecol. 35, 535–545. doi: 10.1016/j.apsoil.2006.09.014
Wu, X., Rensing, C., Han, D., Xiao, K.-Q., Dai, Y., Tang, Z., et al. (2022). Genome-resolved metagenomics reveals distinct phosphorus acquisition strategies between soil microbiomes. Msystems 7:e01107. doi: 10.1128/msystems.01107-21
Wu, Z., Dijkstra, P., Koch, G. W., Peñuelas, J., and Hungate, B. A. (2011). Responses of terrestrial ecosystems to temperature and precipitation change: a meta-analysis of experimental manipulation. Glob. Change Biol. 17, 927–942. doi: 10.1111/j.1365-2486.2010.02302.x
Xiao, W., Chen, X., Jing, X., and Zhu, B. (2018). A meta-analysis of soil extracellular enzyme activities in response to global change. Soil Biol. Biochem. 123, 21–32. doi: 10.1016/j.soilbio.2018.05.001
Xu, G., Chen, J., Berninger, F., Pumpanen, J., Bai, J., Yu, L., et al. (2015). Labile, recalcitrant, microbial carbon and nitrogen and the microbial community composition at two Abies faxoniana forest elevations under elevated temperatures. Soil Biol. Biochem. 91, 1–13. doi: 10.1016/j.soilbio.2015.08.016
Xu, M., Li, X., Kuyper, T. W., Xu, M., Li, X., and Zhang, J. (2021). High microbial diversity stabilizes the responses of soil organic carbon decomposition to warming in the subsoil on the Tibetan Plateau. Glob. Change Biol. 27, 2061–2075. doi: 10.1111/gcb.15553
Xu, Y., Wang, H., Wang, Y., Han, Y., Jiang, Y., and Zhu, W. (2014). Correlation between soil nutrient availability and bacteria community succession in poplar plantations. Chin. J. Appl. Environ. Biol. 20, 491–498.
Xu, Z., Zhang, T., Wang, S., and Wang, Z. (2020). Soil pH and C/N ratio determines spatial variations in soil microbial communities and enzymatic activities of the agricultural ecosystems in Northeast China: Jilin Province case. Appl. Soil Ecol. 155:103629. doi: 10.1016/j.apsoil.2020.103629
Yan, L., Chen, S., Huang, J., and Lin, G. (2011). Water regulated effects of photosynthetic substrate supply on soil respiration in a semiarid steppe. Glob. Change Biol. 17, 1990–2001. doi: 10.1111/j.1365-2486.2010.02365.x
Yang, S., Xu, Z., Wang, R., Zhang, Y., Yao, F., Zhang, Y., et al. (2017). Variations in soil microbial community composition and enzymatic activities in response to increased N deposition and precipitation in inner Mongolian grassland. Appl. Soil Ecol. 119, 275–285. doi: 10.1016/j.apsoil.2017.06.041
Yang, S. L., Zhang, J., and Xu, X. (2007). Influence of the three Gorges Dam on downstream delivery of sediment and its environmental implications. Yangtze River. Geophys. Res. Lett. 34:65. doi: 10.1029/2007GL029472
Yang, Y., Saand, M. A., Huang, L., Abdelaal, W. B., Zhang, J., Wu, Y., et al. (2021). Applications of multi-omics technologies for crop improvement. Front. Plant Sci. 12:563953. doi: 10.3389/fpls.2021.563953
Yuste, J. C., Penuelas, J., Estiarte, M., Garcia-Mas, J., Mattana, S., Ogaya, R., et al. (2011). Drought-resistant fungi control soil organic matter decomposition and its response to temperature. Glob. Change Biol. 17, 1475–1486. doi: 10.1111/j.1365-2486.2010.02300.x
Zak, D. R., Pregitzer, K. S., Curtis, P. S., Teeri, J. A., Fogel, R., and Randlett, D. L. (1993). Elevated atmospheric CO 2 and feedback between carbon and nitrogen cycles. Plant Soil 151, 105–117. doi: 10.1007/BF00010791
Zak, D. R., Tilman, D., Parmenter, R. R., Rice, C. W., Fisher, F. M., Vose, J., et al. (1994). Plant production and soil microorganisms in late-successional ecosystems: a continental-scale study. Ecology 75, 2333–2347. doi: 10.2307/1940888
Zeglin, L., Bottomley, P. J., Jumpponen, A., Rice, C., Arango, M., Lindsley, A., et al. (2013). Altered precipitation regime affects the function and composition of soil microbial communities on multiple time scales. Ecology 94, 2334–2345. doi: 10.1890/12-2018.1
Zelles, L. (1999). Fatty acid patterns of phospholipids and lipopolysaccharides in the characterisation of microbial communities in soil: a review. Biology Fertility Soils 29, 111–129. doi: 10.1007/s003740050533
Zhang, H., Liu, H., Zhao, J., Wang, L., Li, G., Huangfu, C., et al. (2017). Elevated precipitation modifies the relationship between plant diversity and soil bacterial diversity under nitrogen deposition in Stipa baicalensis steppe. Appl. Soil Ecol. 119, 345–353. doi: 10.1016/j.apsoil.2017.06.005
Zhang, N., Liu, W., Yang, H., Yu, X., Gutknecht, J. L., Zhang, Z., et al. (2013). Soil microbial responses to warming and increased precipitation and their implications for ecosystem C cycling. Oecologia 173, 1125–1142. doi: 10.1007/s00442-013-2685-9
Zhou, C., Zhou, G., Zhang, D., Wang, Y., and Liu, S. (2005). CO2 efflux from different forest soils and impact factors in Dinghu Mountain, China. Sci. China Ser. D Earth Sci. 48, 198–206.
Zhou, G., Guan, L., Wei, X., Tang, X., Liu, S., Liu, J., et al. (2008). Factors influencing leaf litter decomposition: an intersite decomposition experiment across China. Plant Soil 311, 61–72. doi: 10.1007/s11104-008-9658-5
Zhou, G., Wang, Y., and Wang, S. (2002). Responses of grassland ecosystems to precipitation and land use along the Northeast China Transect. J. Veg. Sci. 13, 361–368. doi: 10.1111/j.1654-1103.2002.tb02060.x
Zhou, G., Wei, X., Wu, Y., Liu, S., Huang, Y., Yan, J., et al. (2011). Quantifying the hydrological responses to climate change in an intact forested small watershed in S outhern C hina. Glob. Change Biol. 17, 3736–3746. doi: 10.1111/j.1365-2486.2011.02499.x
Zhou, S.-X., Han, B.-H., Xiao, Y.-X., Tang, J.-D., Xiang, Y.-B., and Luo, C. (2017). Simulated nitrogen deposition significantly suppresses the decomposition of forest litter in a natural evergreen broad-leaved forest in the Rainy Area of Western China. Plant Soil 420, 135–145. doi: 10.1007/s11104-017-3383-x
Keywords: edaphic factors, soil metabolites, Picea abies L. stands, precipitation, soil microbial communities, phospholipid fatty acids (PLFA), soil texture
Citation: Chakraborty A, Zádrapová D, Dvořák J, Faltinová Z, Žáček P, Cajthaml T, Korecký J and Roy A (2023) Impact of 30 years precipitation regime differences on forest soil physiology and microbial assemblages. Front. For. Glob. Change 6:1142979. doi: 10.3389/ffgc.2023.1142979
Received: 12 January 2023; Accepted: 22 May 2023;
Published: 13 June 2023.
Edited by:
Maryam Teimouri, Research Institute of Forests and Rangelands, IranReviewed by:
Warren Dick, The Ohio State University, United StatesJianfeng Liu, Chinese Academy of Forestry, China
Copyright © 2023 Chakraborty, Zádrapová, Dvořák, Faltinová, Žáček, Cajthaml, Korecký and Roy. This is an open-access article distributed under the terms of the Creative Commons Attribution License (CC BY). The use, distribution or reproduction in other forums is permitted, provided the original author(s) and the copyright owner(s) are credited and that the original publication in this journal is cited, in accordance with accepted academic practice. No use, distribution or reproduction is permitted which does not comply with these terms.
*Correspondence: Amrita Chakraborty, chakraborty@fld.czu.cz; Amit Roy, roy@fld.czu.cz
†These authors have contributed equally to this work